Pavement crack rapid extraction method based on two-step convolutional neural network
A technology of convolutional neural network and extraction method, which is applied in the field of rapid extraction of pavement cracks based on two-step convolutional neural network, can solve problems such as time and hardware cost infeasible, and achieve long computing time, shortened time-consuming, and time-consuming short effect
- Summary
- Abstract
- Description
- Claims
- Application Information
AI Technical Summary
Problems solved by technology
Method used
Image
Examples
Embodiment Construction
[0036] Below in conjunction with accompanying drawing and specific embodiment the present invention is described in further detail:
[0037] The present invention provides a method for quickly extracting pavement cracks based on a two-step convolutional neural network. Through the two-step convolutional neural network, in the first step, the classification method is used to quickly exclude non-crack areas, and in the second step, the retained Segmentation of suspected crack regions. This method avoids wasting the computing power of the image segmentation model on the non-crack area, greatly speeds up the segmentation efficiency, and realizes the rapid extraction of road surface cracks under the condition of a small loss of accuracy.
[0038] The present invention is based on the two-step convolutional neural network rapid extraction method for pavement cracks, and the specific steps are as follows:
[0039] Step 1: Preprocessing. For the pavement disease images collected by ...
PUM
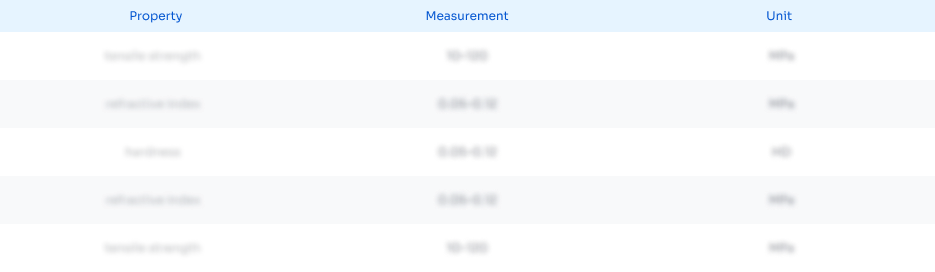
Abstract
Description
Claims
Application Information
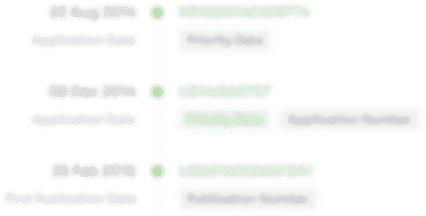
- R&D Engineer
- R&D Manager
- IP Professional
- Industry Leading Data Capabilities
- Powerful AI technology
- Patent DNA Extraction
Browse by: Latest US Patents, China's latest patents, Technical Efficacy Thesaurus, Application Domain, Technology Topic, Popular Technical Reports.
© 2024 PatSnap. All rights reserved.Legal|Privacy policy|Modern Slavery Act Transparency Statement|Sitemap|About US| Contact US: help@patsnap.com