Face synthesis using generative adversarial networks
A face and network technology, applied in the generation of 2D images, acquisition/recognition of facial features, computer parts, etc., can solve problems such as expensive, cumbersome systems, and difficult to obtain.
- Summary
- Abstract
- Description
- Claims
- Application Information
AI Technical Summary
Problems solved by technology
Method used
Image
Examples
Embodiment Construction
[0012] The present disclosure relates to various systems, methods, and computer-readable media for training generative adversarial networks (GANs) for use in facial recognition and training facial recognition networks. Typically, an image of a specific face is fed into a facial recognition network to obtain facial patterns. This face pattern is then input to the GAN generator along with noise values to generate a set of output images. The output images are then fed into a GAN discriminator that uses a database of images to determine a likelihood value indicating that each output image includes a face. Feedback is then sent from the discriminator to the generator, and from the generator to the discriminator, to represent the adversarial losses between the two and to modify the operations of the generator and discriminator to compensate for these adversarial losses. Traditional GANs fail to preserve the identity of a specific face in the output image.
[0013] However, in th...
PUM
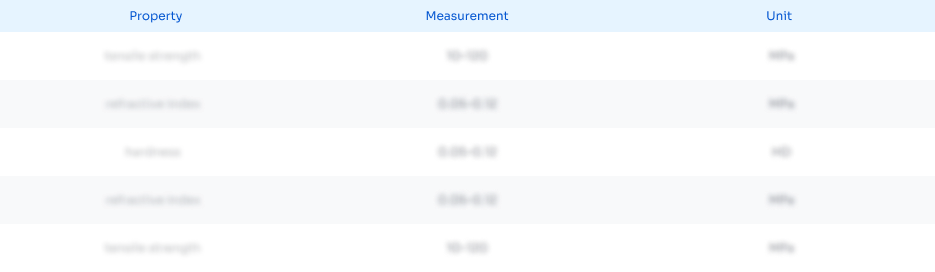
Abstract
Description
Claims
Application Information
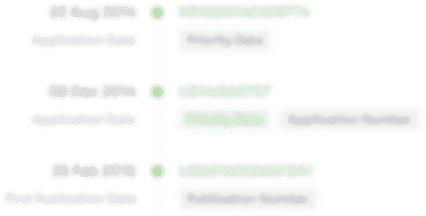
- R&D Engineer
- R&D Manager
- IP Professional
- Industry Leading Data Capabilities
- Powerful AI technology
- Patent DNA Extraction
Browse by: Latest US Patents, China's latest patents, Technical Efficacy Thesaurus, Application Domain, Technology Topic, Popular Technical Reports.
© 2024 PatSnap. All rights reserved.Legal|Privacy policy|Modern Slavery Act Transparency Statement|Sitemap|About US| Contact US: help@patsnap.com