Balanced binarization neural network quantification method and system
A binary neural and neural network technology, applied in neural learning methods, biological neural network models, neural architectures, etc., can solve the problem of model storage consumption and consumption not being well handled, and achieve improved classification performance and activation quantization loss Minimize and reduce the effect of quantization loss
- Summary
- Abstract
- Description
- Claims
- Application Information
AI Technical Summary
Problems solved by technology
Method used
Image
Examples
Embodiment Construction
[0050] The technical content of the present invention will be described in detail below in conjunction with the accompanying drawings and specific embodiments.
[0051] Quantization-based neural network compression acceleration methods can represent weights and activations in the network with very low precision, and the extreme case of quantizing weights and activations to one-bit values can enable neural networks to effectively implement traditional convolution operations through bit-by-bit operations. , enabling small storage and fast inference. The full binarization of the convolutional neural network model can minimize the storage occupation of the model and the amount of calculation of the model, greatly saving the storage space of the parameters, and at the same time convert the calculation of the original parameters from floating-point operations to bit operations, which is very convenient. Dadi speeds up the inference process of the neural network and reduces the amo...
PUM
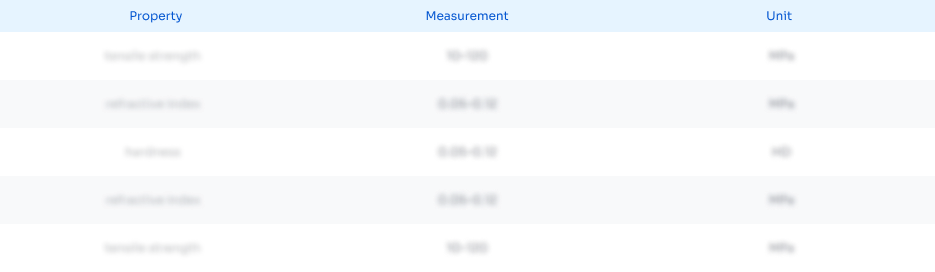
Abstract
Description
Claims
Application Information
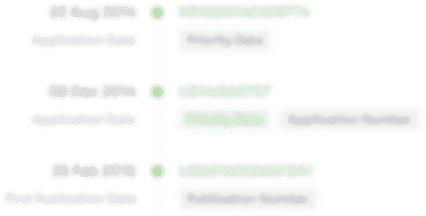
- R&D Engineer
- R&D Manager
- IP Professional
- Industry Leading Data Capabilities
- Powerful AI technology
- Patent DNA Extraction
Browse by: Latest US Patents, China's latest patents, Technical Efficacy Thesaurus, Application Domain, Technology Topic, Popular Technical Reports.
© 2024 PatSnap. All rights reserved.Legal|Privacy policy|Modern Slavery Act Transparency Statement|Sitemap|About US| Contact US: help@patsnap.com