A Sensitive Long Short-Term Memory Method Based on State Change Differentiation
A long-term and short-term memory, state change technology, applied in neural learning methods, neural architecture, biological neural network models, etc., can solve problems such as the inability to achieve real-time performance, and achieve perfect real-time analysis, improve real-time performance, and increase responsiveness. Effect
- Summary
- Abstract
- Description
- Claims
- Application Information
AI Technical Summary
Problems solved by technology
Method used
Image
Examples
Embodiment Construction
[0031] Below in conjunction with accompanying drawing, the technical scheme of the present invention is described in further detail:
[0032] The principle of the invention is as follows: the core of the LSTM neural network is to add a memory module to learn the current information and extract the information and laws associated with the data, so as to transmit the information. A neural unit of the LSTM neural network contains three structures: the input gate i t , forget gate f t and the output gate o t , each step t and its corresponding input sequence are X={x 1 , x 2 ,...,x t }. In order to improve its ability to respond to short-time information, the present invention adds an input differential sequence similar to the state differential effect
[0033] The present invention is a neural unit of a long short-term memory network with increased information sensitivity. The status information of the previous node is obtained from the input terminal c t-1 Input, every...
PUM
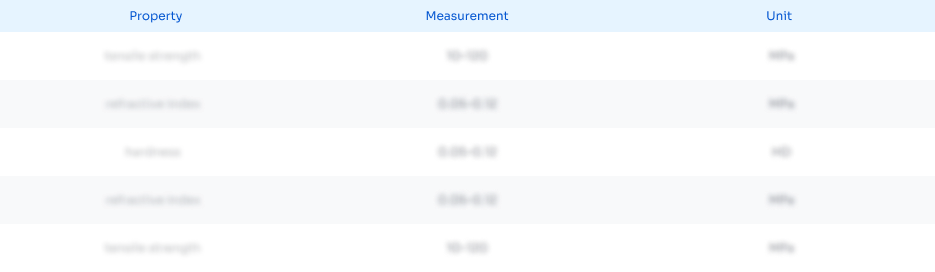
Abstract
Description
Claims
Application Information
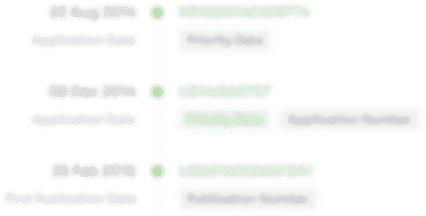
- R&D Engineer
- R&D Manager
- IP Professional
- Industry Leading Data Capabilities
- Powerful AI technology
- Patent DNA Extraction
Browse by: Latest US Patents, China's latest patents, Technical Efficacy Thesaurus, Application Domain, Technology Topic, Popular Technical Reports.
© 2024 PatSnap. All rights reserved.Legal|Privacy policy|Modern Slavery Act Transparency Statement|Sitemap|About US| Contact US: help@patsnap.com