No-reference image quality evaluation method based on multi-branch similarity network
A reference image and quality evaluation technology, applied in the field of image processing, can solve the problems of difficult adaptive representation of distorted image quality, low quality score and subjective consistency, and weak statistical feature expression ability, so as to reduce correlation and improve Versatility, accurate results
- Summary
- Abstract
- Description
- Claims
- Application Information
AI Technical Summary
Benefits of technology
Problems solved by technology
Method used
Image
Examples
Embodiment Construction
[0033] The present invention will be further described below in conjunction with the accompanying drawings and simulation experiments.
[0034] Refer to attached figure 1 , to further describe in detail the specific steps of the present invention.
[0035] Step 1, build a multi-branch convolution module.
[0036] Build a multi-branch convolutional module consisting of the first 4 units of the Inception v4 network.
[0037] The first 4 unit parameters of the Inception v4 network pre-trained on the ImageNet dataset are set as the initialization parameters of each layer of the multi-branch convolution module.
[0038]Step 2, build similarity fusion module.
[0039] Construct a similarity fusion module containing 3 convolution branches and 1 pooling branch.
[0040] The parameter setting of described multi-branch similarity module is as follows:
[0041] The first branch and the fourth branch respectively contain a feature map with a total of 96, a convolution kernel size of ...
PUM
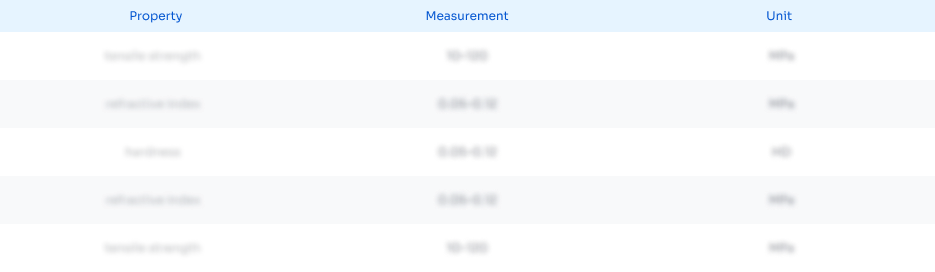
Abstract
Description
Claims
Application Information
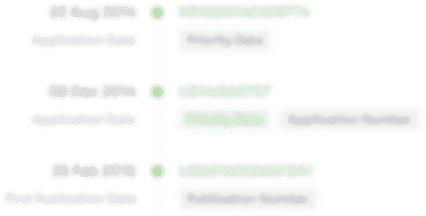
- R&D Engineer
- R&D Manager
- IP Professional
- Industry Leading Data Capabilities
- Powerful AI technology
- Patent DNA Extraction
Browse by: Latest US Patents, China's latest patents, Technical Efficacy Thesaurus, Application Domain, Technology Topic, Popular Technical Reports.
© 2024 PatSnap. All rights reserved.Legal|Privacy policy|Modern Slavery Act Transparency Statement|Sitemap|About US| Contact US: help@patsnap.com