Fault identification method based on Unet + + convolutional neural network
A convolutional neural network and fault identification technology, applied in the field of seismic interpretation, achieves high resolution, good noise resistance, and improved identification accuracy
- Summary
- Abstract
- Description
- Claims
- Application Information
AI Technical Summary
Problems solved by technology
Method used
Image
Examples
Embodiment Construction
[0033] For the convenience of those skilled in the art to understand the technical contents of the present invention, the following technical terms are now explained:
[0034] 1. Convolutional neural network
[0035] CNN can be roughly divided into input layer, hidden layer (including convolution layer, pooling layer and fully connected layer) and output layer. The convolution kernel that can extract different features is the main component of the convolution layer. Each convolution kernel has a fixed-size receptive field. In the receptive field, the volume between the convolution kernel and the input data (the previous layer) is calculated. It can learn the local features of the image. A pooling layer (Pooling Layer) is added between the convolutional layers to downsample, that is, to reduce the size of the features by a certain ratio and perform feature selection and information filtering on the output feature map to reduce the number of model parameters. At the end of the...
PUM
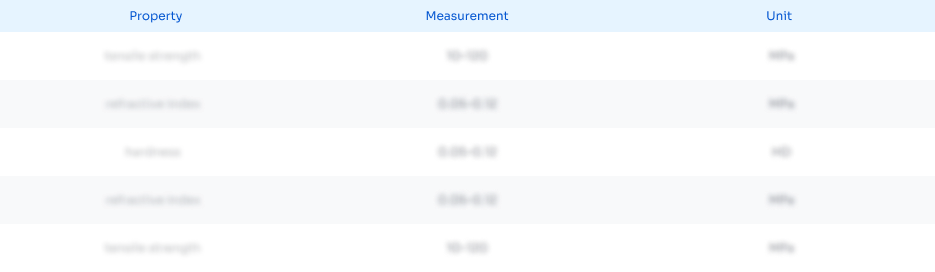
Abstract
Description
Claims
Application Information
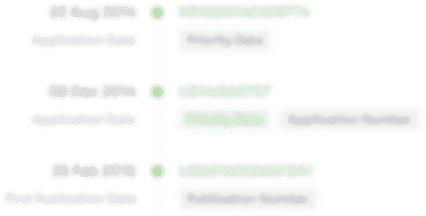
- R&D
- Intellectual Property
- Life Sciences
- Materials
- Tech Scout
- Unparalleled Data Quality
- Higher Quality Content
- 60% Fewer Hallucinations
Browse by: Latest US Patents, China's latest patents, Technical Efficacy Thesaurus, Application Domain, Technology Topic, Popular Technical Reports.
© 2025 PatSnap. All rights reserved.Legal|Privacy policy|Modern Slavery Act Transparency Statement|Sitemap|About US| Contact US: help@patsnap.com