Bearing life prediction method based on hidden Markov model and transfer learning
A technology of transfer learning and bearing life, applied in the direction of neural learning methods, mechanical bearing testing, calculation models, etc., can solve the problems of time neglect, model promotion ability decline, and distribution differences, so as to improve accuracy and effectiveness, save Labor cost and time effects
- Summary
- Abstract
- Description
- Claims
- Application Information
AI Technical Summary
Problems solved by technology
Method used
Image
Examples
Embodiment
[0050]This embodiment discloses a bearing life prediction method based on hidden Markov model and transfer learning, including the following steps,
[0051] (1) Collect the original signal of the whole life of the rolling bearing;
[0052] (2) Extract 13 time-domain features, 16 time-frequency domain features and 3 trigonometric function features from the original signal to form a feature set; see the following table 1 for specific feature descriptions:
[0053] Table 1 Extracted feature description
[0054]
[0055] Among them, two sets of sensors are respectively located in the X-axis direction and the Y-axis direction to collect the original signal of the whole life of the bearing. The whole life here includes the whole time period from the installation of the bearing to the failure of the bearing. Corresponding to each set of sensors, the above Thirty-two groups of features. In this way, by extracting the degradation information of the characteristic reaction bearing ...
PUM
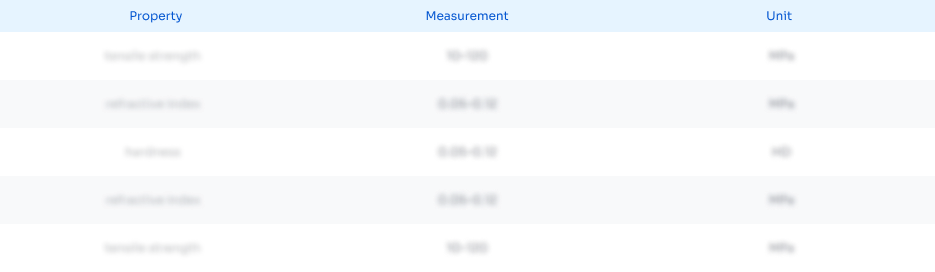
Abstract
Description
Claims
Application Information
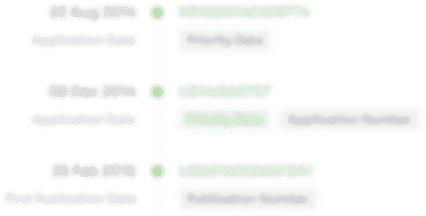
- R&D Engineer
- R&D Manager
- IP Professional
- Industry Leading Data Capabilities
- Powerful AI technology
- Patent DNA Extraction
Browse by: Latest US Patents, China's latest patents, Technical Efficacy Thesaurus, Application Domain, Technology Topic, Popular Technical Reports.
© 2024 PatSnap. All rights reserved.Legal|Privacy policy|Modern Slavery Act Transparency Statement|Sitemap|About US| Contact US: help@patsnap.com