Tumor region segmentation method and system for liver CT image based on cascaded full convolutional network
A fully convolutional network, tumor area technology, applied in image analysis, image enhancement, medical image and other directions, can solve the problem of low image segmentation accuracy, and achieve the effect of improving accuracy and improving accuracy
- Summary
- Abstract
- Description
- Claims
- Application Information
AI Technical Summary
Problems solved by technology
Method used
Image
Examples
Embodiment Construction
[0050] In order to make the object, technical solution and advantages of the present invention clearer, the present invention will be described in detail below with reference to the accompanying drawings and specific embodiments.
[0051] The role of pooling in the full convolutional network is to perform downsampling. Each convolutional layer in the network will be connected to a pooling layer. The role of pooling in the convolutional neural network is to combine these features after the convolutional layer extracts features. Feature points in a small neighborhood are integrated into new features. The purpose of pooling is to reduce features and parameters, but the purpose of pooling is to maintain feature invariance. Commonly used are average pooling, maximum pooling and random pooling. Average pooling is better for background preservation, and maximum pooling It is more conducive to extracting texture. Pooling can reduce the dimension of feature representation and reduce c...
PUM
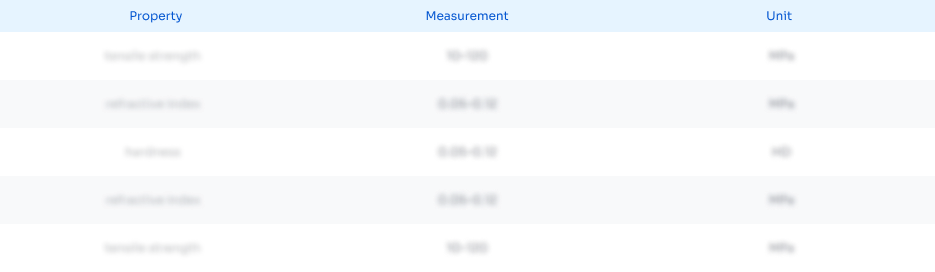
Abstract
Description
Claims
Application Information
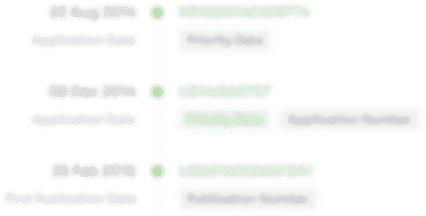
- R&D
- Intellectual Property
- Life Sciences
- Materials
- Tech Scout
- Unparalleled Data Quality
- Higher Quality Content
- 60% Fewer Hallucinations
Browse by: Latest US Patents, China's latest patents, Technical Efficacy Thesaurus, Application Domain, Technology Topic, Popular Technical Reports.
© 2025 PatSnap. All rights reserved.Legal|Privacy policy|Modern Slavery Act Transparency Statement|Sitemap|About US| Contact US: help@patsnap.com