Aluminum electrolysis superheat degree recognition method based on CNN-LapsELM
A technology of aluminum electrolysis and superheating, applied in neural learning methods, character and pattern recognition, image data processing, etc., can solve the problems of human error, waste of manpower and material resources, etc.
- Summary
- Abstract
- Description
- Claims
- Application Information
AI Technical Summary
Problems solved by technology
Method used
Image
Examples
Embodiment 1
[0050] 1. Dataset description and preprocessing
[0051] During the aluminum electrolysis industrial process, fire eye images are collected by operators using industrial camera equipment. Affected by the industrial environment and physical equipment, there are some noises and interferences in the collected fire eye images. Therefore, it is crucial to preprocess the Huoyan image. Here, the adaptive mean filtering algorithm is used to remove the noise points on the original fire eye image, so as to exclude the influence of noise on the classification of superheat state. In addition, because not all of the collected Huoyan images are Huoyan parts, in order to avoid other parts from interfering with the recognition results, improve the processing efficiency of Huoyan images, and reduce the memory burden of CNN when extracting features, it is necessary to preprocess the Huoyan images, from Extract the edge of the fire hole in the whole image.
[0052] The experiment uses 1200 im...
Embodiment 2
[0063] 1. Laplacian regularization:
[0064] Usually, the recognition accuracy of the model trained with a small number of labeled training sample sets does not meet the expected value. In order to solve the problem of few labeled training samples and improve the performance of the model, a semi-supervised learning method is proposed. Given a labeled data set and the unlabeled dataset Then the method based on Laplacian semi-supervised learning can fully extract the geometric information distribution contained in all available data. The semi-supervised learning method is based on the following two basic assumptions:
[0065] (1) All with label X l and unlabeled data X u are drawn from the same marginal distribution.
[0066] (2) If two sample points x 1 and x 2 , the distributions are very close to each other, then the corresponding conditional probability P(y|x 1 ) and P(y|x 2 ) should be very similar.
[0067] Using these two assumptions, the target regularization ...
PUM
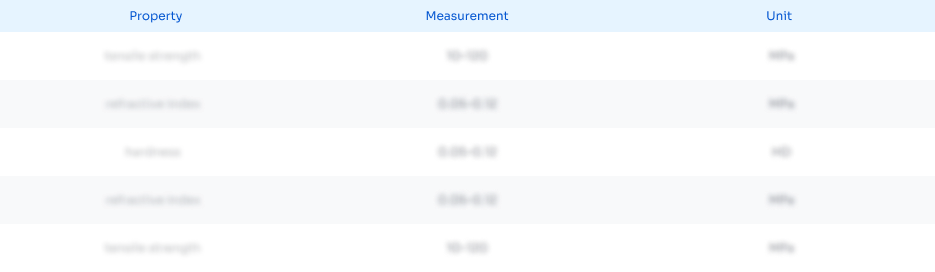
Abstract
Description
Claims
Application Information
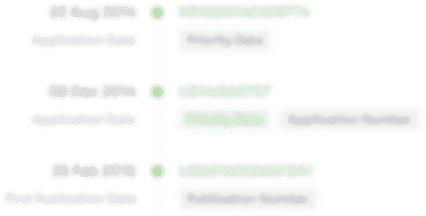
- R&D Engineer
- R&D Manager
- IP Professional
- Industry Leading Data Capabilities
- Powerful AI technology
- Patent DNA Extraction
Browse by: Latest US Patents, China's latest patents, Technical Efficacy Thesaurus, Application Domain, Technology Topic, Popular Technical Reports.
© 2024 PatSnap. All rights reserved.Legal|Privacy policy|Modern Slavery Act Transparency Statement|Sitemap|About US| Contact US: help@patsnap.com