Bearing fault diagnosis method under variable working condition based on mixed entropy and joint distribution adaptation
A technology of joint distribution and fault diagnosis, applied in the testing of mechanical components, testing of machine/structural components, instruments, etc., can solve problems such as the inability to meet accurate bearing diagnosis, intelligent maintenance, lack of bearing fault diagnosis methods, etc., and achieve a reliable theory. Guidance and technical support, the effect of improving accuracy
- Summary
- Abstract
- Description
- Claims
- Application Information
AI Technical Summary
Problems solved by technology
Method used
Image
Examples
Embodiment Construction
[0044] The technical solutions of the present invention will be described in detail below in conjunction with the accompanying drawings.
[0045] In order to make the object, technical solution and advantages of the present invention clearer, the present invention will be further described in detail below in conjunction with the accompanying drawings and embodiments. It should be understood that the specific embodiments described here are only used to explain the present invention, not to limit the present invention. In addition, the technical features involved in the various embodiments of the present invention described below can be combined with each other as long as they do not constitute a conflict with each other.
[0046] Such as figure 1 As shown, the present invention proposes a bearing fault diagnosis method under variable working conditions based on mixed entropy and joint distribution adaptation, and the steps are as follows.
[0047] Step 1: divide the original ...
PUM
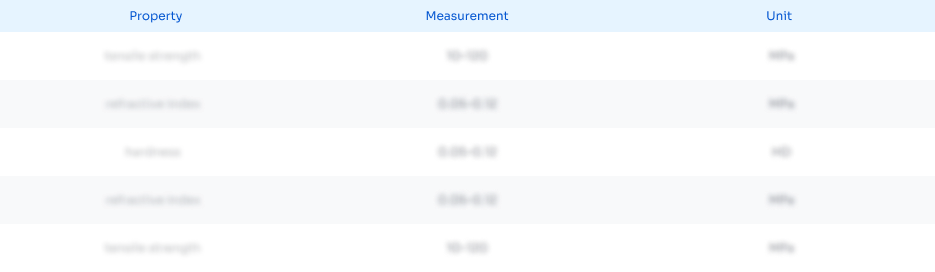
Abstract
Description
Claims
Application Information
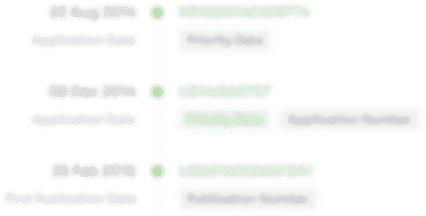
- R&D Engineer
- R&D Manager
- IP Professional
- Industry Leading Data Capabilities
- Powerful AI technology
- Patent DNA Extraction
Browse by: Latest US Patents, China's latest patents, Technical Efficacy Thesaurus, Application Domain, Technology Topic, Popular Technical Reports.
© 2024 PatSnap. All rights reserved.Legal|Privacy policy|Modern Slavery Act Transparency Statement|Sitemap|About US| Contact US: help@patsnap.com