Aluminum profile defect detection method based on improved Faster-RCNN
A defect detection and aluminum profile technology, applied in the field of computer vision, can solve problems such as low efficiency and poor reliability
- Summary
- Abstract
- Description
- Claims
- Application Information
AI Technical Summary
Problems solved by technology
Method used
Image
Examples
Embodiment Construction
[0078] The technical solution of the present invention will be specifically described below in conjunction with the accompanying drawings.
[0079] Such as figure 1 As shown, an aluminum profile defect detection method based on the improved Faster-RCNN includes the following steps:
[0080] Step S1: Create a data set of aluminum profile defects in VOC2007 format:
[0081] The step S1 specifically includes:
[0082] Step S11: download the VOC2007 dataset, and move the dataset to the data folder;
[0083] Step S12: For the samples of the aluminum profile defect image data set, analyze the distribution of the samples, and perform data enhancement on a small number of samples, including methods of mirroring, rotation, cropping, translation, adding Gaussian noise, and adjusting image brightness and saturation Perform data augmentation and move the pictures to the JPEGImages folder;
[0084] Step S13: Use the annotation tool to annotate the data set, including the name of the im...
PUM
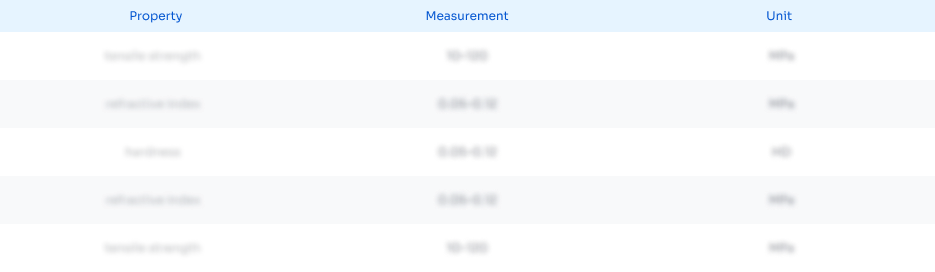
Abstract
Description
Claims
Application Information
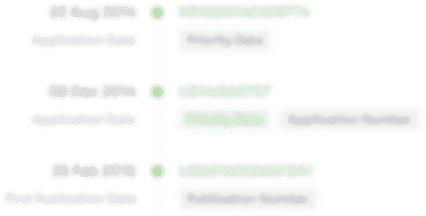
- R&D
- Intellectual Property
- Life Sciences
- Materials
- Tech Scout
- Unparalleled Data Quality
- Higher Quality Content
- 60% Fewer Hallucinations
Browse by: Latest US Patents, China's latest patents, Technical Efficacy Thesaurus, Application Domain, Technology Topic, Popular Technical Reports.
© 2025 PatSnap. All rights reserved.Legal|Privacy policy|Modern Slavery Act Transparency Statement|Sitemap|About US| Contact US: help@patsnap.com