Aerial image segmentation method based on global and multi-scale full convolutional networks
A fully convolutional network and aerial image technology, which is applied in the field of aerial image segmentation, can solve the problems of not considering the different functions of the decoding part of the full convolution network, not considering the simplicity and efficiency of the network, and the low performance of network segmentation, so as to achieve robustness High, overcome simplicity and high efficiency, and reduce the effect of high-frequency information loss
- Summary
- Abstract
- Description
- Claims
- Application Information
AI Technical Summary
Problems solved by technology
Method used
Image
Examples
Embodiment Construction
[0037] The present invention will be further described in detail below in conjunction with the accompanying drawings.
[0038] Reference attached figure 1 , The implementation steps of the present invention will be further described in detail.
[0039] Step 1. Construct a global and multi-scale full convolutional network.
[0040] The first step is to build a global and multi-scale full convolutional network. Its structure is: input layer → feature extraction layer → first combination module → fully connected layer → deconvolution layer → second combination module → output layer.
[0041] The feature extraction layer is a VGG16 model composed of five convolution modules connected in series.
[0042] The first combination module has 7 layers, and its structure is in order: first convolution layer→transpose layer→first multiplication layer→softmax layer→second multiplication layer→second convolution layer→addition layer.
[0043] The structure of the fully connected layer is in order: maxi...
PUM
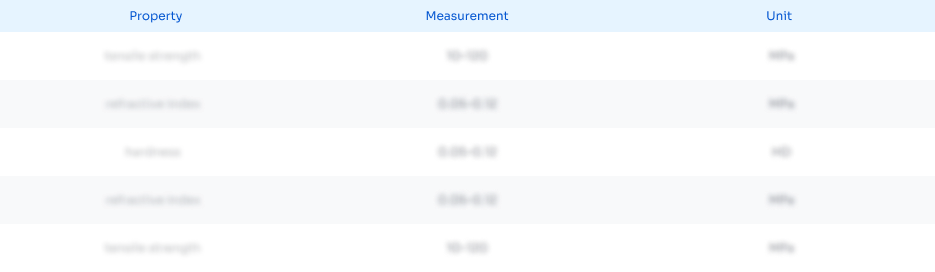
Abstract
Description
Claims
Application Information
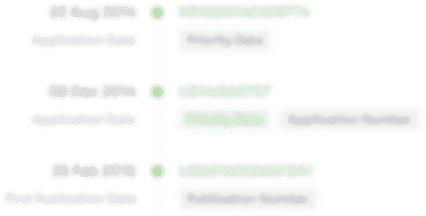
- R&D Engineer
- R&D Manager
- IP Professional
- Industry Leading Data Capabilities
- Powerful AI technology
- Patent DNA Extraction
Browse by: Latest US Patents, China's latest patents, Technical Efficacy Thesaurus, Application Domain, Technology Topic, Popular Technical Reports.
© 2024 PatSnap. All rights reserved.Legal|Privacy policy|Modern Slavery Act Transparency Statement|Sitemap|About US| Contact US: help@patsnap.com