Training and tracking method based on multi-challenge perception learning model
A technology of perceptual learning and training methods, applied in neural learning methods, biological neural network models, neural architectures, etc., can solve problems such as poor extraction of challenge information, achieve the effects of increasing richness, real-time tracking performance, and ensuring accuracy
- Summary
- Abstract
- Description
- Claims
- Application Information
AI Technical Summary
Problems solved by technology
Method used
Image
Examples
Embodiment 1
[0053] like figure 1 , figure 1 It is a schematic diagram of the structure of the network model; the training method based on the multi-challenge perception learning model includes the following steps;
[0054] S11. Constructing a network model;
[0055] Obtain the first frame of the current tracking video sequence, and use the center point of the truth frame as the mean value to carry out Gaussian distribution sampling to obtain candidate samples by the truth frame of the target in the given first frame. In this embodiment, (0.09r 2 ,0.09r 2 ,0.25) is the covariance, resulting in 256 candidate samples;
[0056] Among them: r is the average value of the width and height of the target in the previous frame,
[0057] Obtaining the current tracking video sequence is an existing technology, such as obtaining through a camera, etc., and will not be described in detail here, and Gaussian distribution sampling is also an existing technology.
[0058] like figure 2 , the networ...
Embodiment 2
[0081] like figure 1 , image 3 , Figure 4 , figure 1 It is a schematic diagram of the network model structure; image 3 It is a flow block diagram of embodiment 2 in the present invention; Figure 4 is a flowchart of the real-time visual tracking method based on the multi-challenge perceptual learning model;
[0082] A real-time visual tracking method based on a multi-challenge perceptual learning model, comprising the following steps;
[0083] S21. Input the currently tracked video frame, and use Gaussian sampling to obtain candidate samples of the current frame around the target position predicted in the previous frame;
[0084] The first frame image provided by the video sequence to be tracked is used as the previous frame; 5500 samples are randomly generated according to the Gaussian distribution from the previous frame and the ground-truth box that frames the target location area, S + =500(IOU≥0.7) and S - =5000(IOU≤0.3);
[0085] Use 5500 samples to initialize ...
PUM
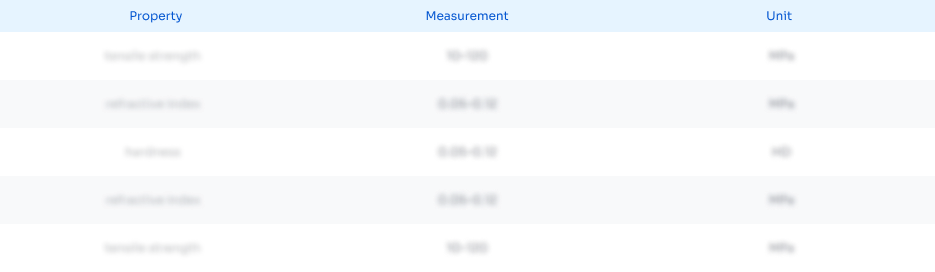
Abstract
Description
Claims
Application Information
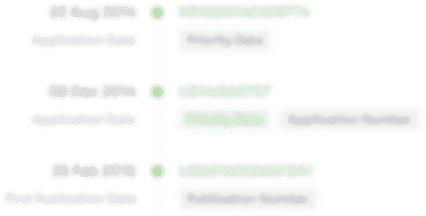
- R&D Engineer
- R&D Manager
- IP Professional
- Industry Leading Data Capabilities
- Powerful AI technology
- Patent DNA Extraction
Browse by: Latest US Patents, China's latest patents, Technical Efficacy Thesaurus, Application Domain, Technology Topic, Popular Technical Reports.
© 2024 PatSnap. All rights reserved.Legal|Privacy policy|Modern Slavery Act Transparency Statement|Sitemap|About US| Contact US: help@patsnap.com