Network structure deanonymization system and method based on matrix decomposition
A network structure and matrix decomposition technology, applied in transmission systems, digital transmission systems, data exchange networks, etc., can solve problems such as limited accuracy, global structural information is not widely used, etc., to prevent inference attacks.
- Summary
- Abstract
- Description
- Claims
- Application Information
AI Technical Summary
Problems solved by technology
Method used
Image
Examples
Embodiment 1
[0044] In this embodiment, the present invention proposes a network structure deanonymization system based on matrix decomposition, including a dynamic network acquisition data module, a low-rank sparse model training module, a non-negative matrix decomposition training model building module, an optimization module, in:
[0045] The dynamic network-static network conversion module is used to obtain the adjacency matrix of the static network and the static network according to the social network data of the real-time dynamic network;
[0046] The low-rank sparse model training module is used to constrain the local feature structure of each static network while removing the noise of each static network;
[0047] The building block of the non-negative matrix factorization training model, which is used to capture the inherent laws of the network and describe the potential characteristics of the network;
[0048] The optimization module is used to reduce the randomness of the netw...
Embodiment 2
[0065] This embodiment proposes a network structure deanonymization method based on matrix decomposition, such as figure 1 , including the following steps:
[0066] S1. Acquire the data source, the data source includes the topology structure of the relationship between various networks and the topology structure of the relationship between users in the network; that is, figure 2 The dynamic network shown, for example, the friendship topology on Tencent Weibo may be similar to the friendship topology on Facebook. These two networks have some common users, that is, the topology of the relationship between the networks, and the relationship between users in the network. The topology of the relationship is the topology of the relationship between users in a network;
[0067] S2, such as figure 2 , divide the data set of the dynamic social network into T static network data sets according to time, and obtain the adjacency matrix corresponding to each static network structure in...
Embodiment 3
[0085] As an optional implementation, the solution process of the target matrix includes:
[0086]
[0087] Among them, rank(S t ) means; λ means the damping coefficient; E t Represents the noise error matrix of the t-th static network; A t Represents the adjacency matrix of the t-th static network; S t Represents the target matrix of the tth static network; ||E t || 0 Represents the sparse noise constraint; S represents the target matrix after low-rank sparse model training; E represents the noise error matrix of the network.
PUM
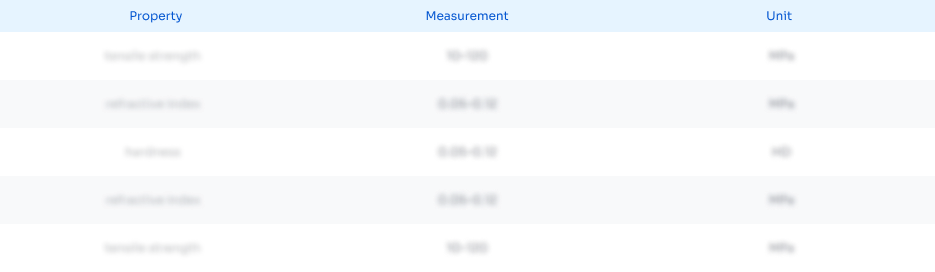
Abstract
Description
Claims
Application Information
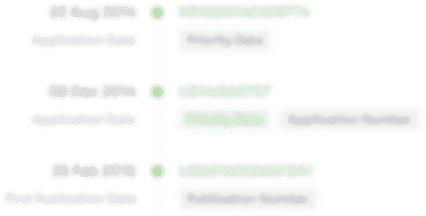
- R&D
- Intellectual Property
- Life Sciences
- Materials
- Tech Scout
- Unparalleled Data Quality
- Higher Quality Content
- 60% Fewer Hallucinations
Browse by: Latest US Patents, China's latest patents, Technical Efficacy Thesaurus, Application Domain, Technology Topic, Popular Technical Reports.
© 2025 PatSnap. All rights reserved.Legal|Privacy policy|Modern Slavery Act Transparency Statement|Sitemap|About US| Contact US: help@patsnap.com