Fault diagnosis method based on minimum entropy deconvolution and stacked sparse auto-encoder
A sparse self-encoder and fault diagnosis technology, applied in the testing of machine/structural components, instruments, and mechanical components, etc., can solve cumbersome problems, achieve high fault recognition, high recognition rate, and solve cumbersome and time-consuming problems. the effect of time problems
- Summary
- Abstract
- Description
- Claims
- Application Information
AI Technical Summary
Problems solved by technology
Method used
Image
Examples
Embodiment Construction
[0067] The present invention will be further described below in conjunction with the accompanying drawings and embodiments.
[0068] In this embodiment, the fault diagnosis method based on minimum entropy deconvolution and stacked sparse autoencoder includes the following steps:
[0069] Step 1-1: Collect the original fault vibration signal of the object to be diagnosed;
[0070] Step 1-2: Denoise the original fault vibration signal through minimum entropy deconvolution to obtain fault samples;
[0071] Steps 1-3: divide the fault samples into multiple training samples and test samples;
[0072] Steps 1-4: Using multiple training samples to train the multi-fault classifier based on the stacked sparse denoising autoencoder;
[0073] Steps 1-5: Use the trained multi-fault classifier (stacked sparse autoencoder) to classify the test samples;
[0074] Steps 1-6: Identify the working status and fault type of the object according to the classification results.
[0075] Compared ...
PUM
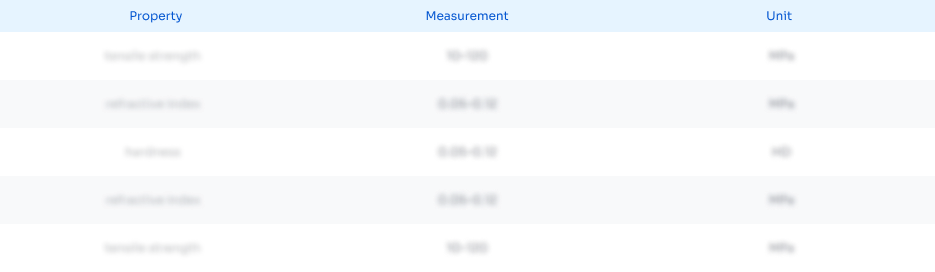
Abstract
Description
Claims
Application Information
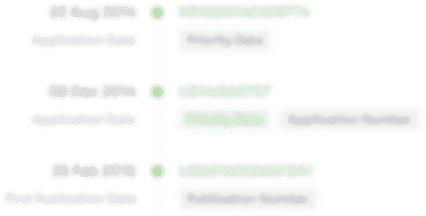
- R&D Engineer
- R&D Manager
- IP Professional
- Industry Leading Data Capabilities
- Powerful AI technology
- Patent DNA Extraction
Browse by: Latest US Patents, China's latest patents, Technical Efficacy Thesaurus, Application Domain, Technology Topic.
© 2024 PatSnap. All rights reserved.Legal|Privacy policy|Modern Slavery Act Transparency Statement|Sitemap