Irregular region flow prediction method based on multi-graph convolution and GRU
A traffic forecasting and irregular technology, applied in forecasting, character and pattern recognition, biological neural network model, etc.
- Summary
- Abstract
- Description
- Claims
- Application Information
AI Technical Summary
Problems solved by technology
Method used
Image
Examples
Embodiment Construction
[0049] The present invention will be specifically introduced below in conjunction with the accompanying drawings and specific embodiments.
[0050] Such as figure 1 As shown, the traffic forecasting method for irregular areas based on multi-image convolution and GRU of the present invention includes the following steps:
[0051] Step 1, divide the city into N unconnected irregular areas;
[0052] Step 2. Simplify the historical trajectory data in time and space, and calculate the inflow and outflow of all regions at each time step;
[0053] Step 3: Establish multiple association graphs between regions, and construct corresponding adjacency matrices to represent the diverse spatial associations between irregular regions;
[0054] Step 4, designing a multi-image convolutional neural network based on the inter-regional correlation graph, fusing diverse spatial correlation features between regions, and obtaining the result of multi-image convolution fusion;
[0055] Step 5, bas...
PUM
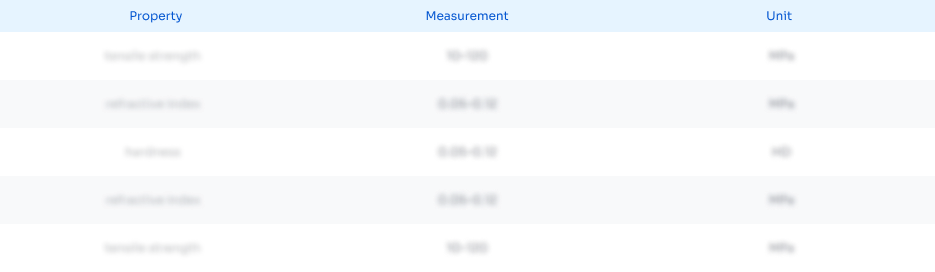
Abstract
Description
Claims
Application Information
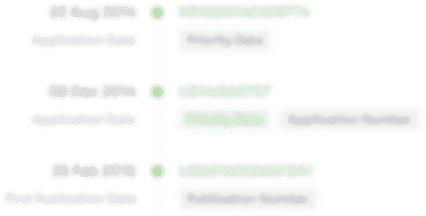
- R&D Engineer
- R&D Manager
- IP Professional
- Industry Leading Data Capabilities
- Powerful AI technology
- Patent DNA Extraction
Browse by: Latest US Patents, China's latest patents, Technical Efficacy Thesaurus, Application Domain, Technology Topic, Popular Technical Reports.
© 2024 PatSnap. All rights reserved.Legal|Privacy policy|Modern Slavery Act Transparency Statement|Sitemap|About US| Contact US: help@patsnap.com