Bearing fault diagnosis method based on deep adversarial migration network
A fault diagnosis and network technology, applied in neural learning methods, biological neural network models, testing of mechanical components, etc., can solve the problem of accurate identification of difficult faults, difficult to adapt and meet the engineering application requirements of mechanical fault intelligent diagnosis, and labeling of working condition data Difficulties and other problems, to achieve the effect of solving difficult and effective diagnosis
- Summary
- Abstract
- Description
- Claims
- Application Information
AI Technical Summary
Problems solved by technology
Method used
Image
Examples
Embodiment Construction
[0027] The specific embodiment of the present invention will be further described below in conjunction with accompanying drawing and specific embodiment:
[0028] A bearing fault diagnosis method based on a deep adversarial migration network, comprising the following steps:
[0029] Step 1, collect samples, set different speed and load conditions of bearings, and collect vibration signals of different faulty bearings under various working conditions to obtain spectrum samples;
[0030] Step 2, build a generator-based feature extraction network: such as figure 2 Shown is the basic structure of a generative adversarial neural network. Establish a deep adversarial migration model based on the generative adversarial neural network, use the labeled data in a certain working condition as the source domain data, and the unlabeled data in other working conditions as the target domain data for model training, through the confrontation between the generator and the discriminator The ...
PUM
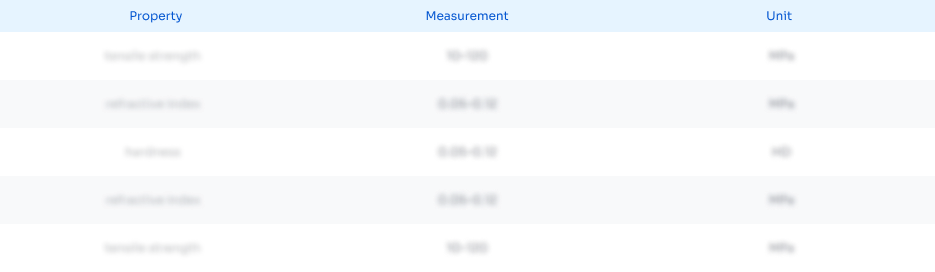
Abstract
Description
Claims
Application Information
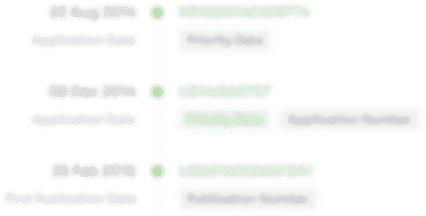
- R&D Engineer
- R&D Manager
- IP Professional
- Industry Leading Data Capabilities
- Powerful AI technology
- Patent DNA Extraction
Browse by: Latest US Patents, China's latest patents, Technical Efficacy Thesaurus, Application Domain, Technology Topic, Popular Technical Reports.
© 2024 PatSnap. All rights reserved.Legal|Privacy policy|Modern Slavery Act Transparency Statement|Sitemap|About US| Contact US: help@patsnap.com