Bearing fault classification method and system based on deep learning network
A technology of deep learning network and fault classification, applied in neural learning methods, biological neural network models, testing of mechanical components, etc., can solve problems such as gradient disappearance, performance degradation, and poor classification effect
- Summary
- Abstract
- Description
- Claims
- Application Information
AI Technical Summary
Problems solved by technology
Method used
Image
Examples
no. 1 example
[0091] Select normal bearings, roller faults, inner ring faults, outer ring faults, outer ring roller compound faults and inner ring roller compound faults. Point faults for bearings in 11 states.
[0092] S1, the collection frequency is set to 20480Hz, (that is, the sampling point data is 20480 points per second), and 8 working conditions are set for rolling bearings (as shown in Table 1), which are 6 steady-state working conditions and 2 For variable speed conditions, F in Table 1 represents the load on the rolling bearing, n represents the speed of the rolling bearing, and n=2000-4000-2000rpm indicates that the speed of the rolling bearing changes from 2000rpm-4000rpm-2000rpm in turn. Collect corresponding vibration signal data for each working condition.
[0093] Table 1
[0094]
[0095] Among them, the change of the rotational speed has an impact on the vibration signal data. For example, a rolling bearing with a single-point fault on the outer ring, such as Figur...
PUM
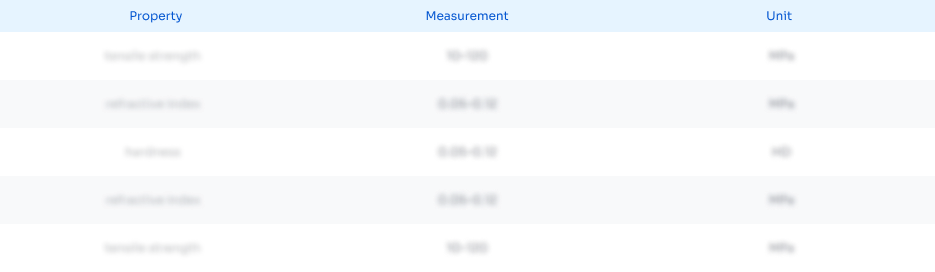
Abstract
Description
Claims
Application Information
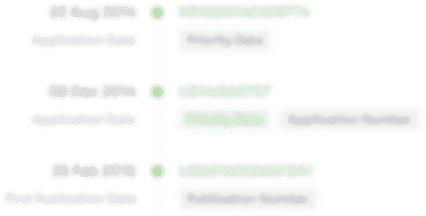
- R&D Engineer
- R&D Manager
- IP Professional
- Industry Leading Data Capabilities
- Powerful AI technology
- Patent DNA Extraction
Browse by: Latest US Patents, China's latest patents, Technical Efficacy Thesaurus, Application Domain, Technology Topic, Popular Technical Reports.
© 2024 PatSnap. All rights reserved.Legal|Privacy policy|Modern Slavery Act Transparency Statement|Sitemap|About US| Contact US: help@patsnap.com