Hoisting process danger identification method and system based on deep learning
A hazard identification and deep learning technology, applied in neural learning methods, character and pattern recognition, biological neural network models, etc., can solve problems such as inability to effectively prevent hoisting accidents, single hazard identification methods, etc. Comprehensive and practical effect
- Summary
- Abstract
- Description
- Claims
- Application Information
AI Technical Summary
Problems solved by technology
Method used
Image
Examples
Embodiment 1
[0054] Such as figure 1 As shown, this embodiment proposes a method for hazard identification in the hoisting process based on deep learning, including:
[0055] S1. Training and generating Faster R-CNN workers and hook detection network;
[0056] In order to improve the efficiency of hazard identification in the hoisting process, the present invention uses Faster R-CNN to detect workers and hooks. The Faster RCNN network is one of the more popular general multi-target detection frameworks. The existing Faster RCNN algorithm is mainly divided into three parts: the first part is the CNN basic network, which is used to complete the extraction of image features; the second part is the RPN (Region Proposal Networks) network, which mainly uses the convolutional neural network to directly generate regions target, the method used is essentially a sliding window. A fixed-size window is used to slide on the last layer feature map of the CNN basic network, and each window will outp...
Embodiment 2
[0079] Such as figure 2 As shown, this embodiment proposes a hazard identification system for hoisting process based on deep learning, including:
[0080] The training module is used to train and generate Faster R-CNN workers and hook detection network;
[0081] In order to improve the efficiency of hazard identification in the hoisting process, the present invention uses Faster R-CNN to detect workers and hooks. The Faster RCNN network is one of the more popular general multi-target detection frameworks. The existing Faster RCNN algorithm is mainly divided into three parts: the first part is the CNN basic network, which is used to complete the extraction of image features; the second part is the RPN (Region Proposal Networks) network, which mainly uses the convolutional neural network to directly generate regions target, the method used is essentially a sliding window. A fixed-size window is used to slide on the last layer feature map of the CNN basic network, and each wi...
PUM
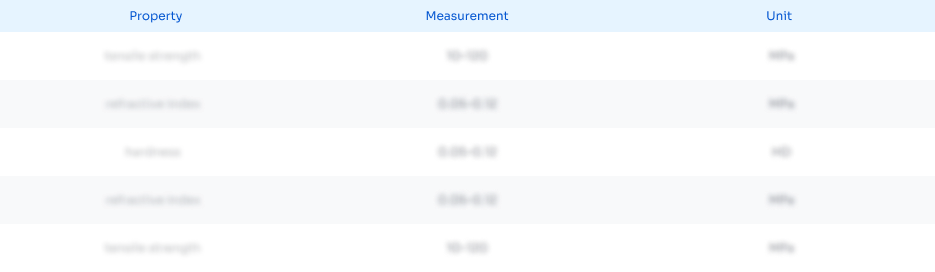
Abstract
Description
Claims
Application Information
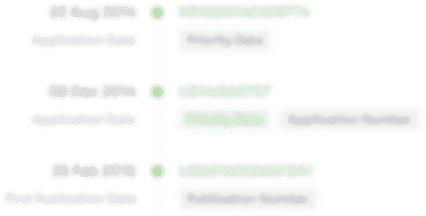
- R&D Engineer
- R&D Manager
- IP Professional
- Industry Leading Data Capabilities
- Powerful AI technology
- Patent DNA Extraction
Browse by: Latest US Patents, China's latest patents, Technical Efficacy Thesaurus, Application Domain, Technology Topic, Popular Technical Reports.
© 2024 PatSnap. All rights reserved.Legal|Privacy policy|Modern Slavery Act Transparency Statement|Sitemap|About US| Contact US: help@patsnap.com