Floor damage fault image identification method
A technology for image recognition and flooring, applied in character and pattern recognition, instruments, biological neural network models, etc., can solve problems such as low accuracy, low efficiency, and high cost, and achieve the goals of improving efficiency, reducing safety hazards, and saving labor costs Effect
- Summary
- Abstract
- Description
- Claims
- Application Information
AI Technical Summary
Problems solved by technology
Method used
Image
Examples
specific Embodiment approach 1
[0025] Specific implementation mode one: the specific process of the floor damage fault image recognition method in this implementation mode is as follows:
[0026] Step 1. Collect samples and create data sets;
[0027] Step 2: Perform preprocessing on the image to be recognized collected by the device, and obtain the image to be recognized after preprocessing; the specific process is:
[0028] Because in the actual detection process, due to the harsh working environment of the truck floor, the collected pictures will produce various noises. When used as training samples, the noise in the pictures will be extracted as feature values, which will seriously interfere with the recognition results. In view of this situation, Gaussian filtering and gray level equalization operations are performed on the collected images to be recognized to remove noise;
[0029] Step 3: Establish a VGG model, use the training set to train the VGG model, and obtain a pre-trained VGG model; the speci...
specific Embodiment approach 2
[0040] Specific embodiment two: the difference between this embodiment and specific embodiment one is that the samples are collected in the step one, and the data set is established; the specific process is:
[0041] Step 11. Use a high-resolution line-scan camera to collect a clear grayscale image of the railway wagon floor; the principle of collecting the image is as follows:
[0042] (1) Collect the floor images of railway wagons under various conditions such as rainwater, ice and snow, chalk graffiti, load leakage, mud stains, oil stains, black paint, and dust;
[0043] (2) Collect floor images of railway wagons at different stations, different equipment, and at different times (different degrees of sunlight interference);
[0044] (3) According to the material of the railway wagon floor, images of wagon floors such as steel floors and wooden floors are collected respectively;
[0045] Step 12. Sample amplification: Due to the limited sample data actually collected, in or...
specific Embodiment approach 3
[0047] Specific embodiment three: the difference between this embodiment and specific embodiment one or two is that the VGG model is established in the step three one; the specific process is:
[0048] VGG model structure such as figure 2 shown;
[0049] VGG model includes input layer, 64-channel conv2 convolutional layer 1, 64-channel conv2 convolutional layer 2, pool_1 pooling layer, 128-channel conv3 convolutional layer 1, 128-channel conv3 convolutional layer 2, pool_2 pooling layer, 256 channels conv4 convolutional layer 1, 256-channel conv4 convolutional layer 2, 256-channel conv4 convolutional layer 3, 256-channel conv4 convolutional layer 4, pool_3 pooling layer, 512-channel conv5 convolutional layer 1, 512-channel conv5 convolutional layer 2 , 512-channel conv5 convolutional layer 3, 512-channel conv5 convolutional layer 4, pool_4 pooling layer, 512-channel conv5 convolutional layer 5, 512-channel conv5 convolutional layer 6, 512-channel conv5 convolutional layer 7,...
PUM
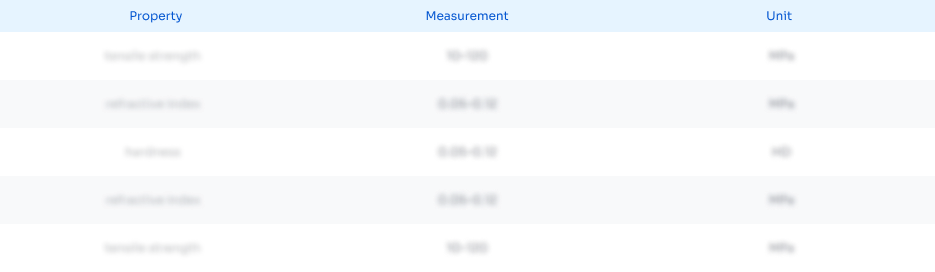
Abstract
Description
Claims
Application Information
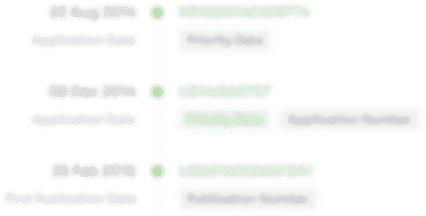
- R&D
- Intellectual Property
- Life Sciences
- Materials
- Tech Scout
- Unparalleled Data Quality
- Higher Quality Content
- 60% Fewer Hallucinations
Browse by: Latest US Patents, China's latest patents, Technical Efficacy Thesaurus, Application Domain, Technology Topic, Popular Technical Reports.
© 2025 PatSnap. All rights reserved.Legal|Privacy policy|Modern Slavery Act Transparency Statement|Sitemap|About US| Contact US: help@patsnap.com