No-reference image quality map generation method based on adversarial generative network
A map generation and reference image technology, which is applied in the field of image processing, can solve the problems of low performance and poor performance of evaluation methods without reference
- Summary
- Abstract
- Description
- Claims
- Application Information
AI Technical Summary
Problems solved by technology
Method used
Image
Examples
Embodiment Construction
[0086] The present invention will be further described below.
[0087] Such as figure 1 As shown, a no-reference image quality map generation method based on the confrontational generative network, firstly, the image must be preprocessed to obtain the similarity map corresponding to the distortion map: SSIM_MAP and FSIM_MAP, and then a neural network framework based on the U-net network is trained , input the distortion map to the trained network, the similarity map of the distortion map can be obtained, and the corresponding quality score can be obtained through the similarity map. The specific implementation steps are as follows:
[0088] Step 1: Preprocessing to get a similar graph
[0089] 1-1. Calculate the similarity map SSIM_MAP
[0090] 1-1-1. Calculate brightness contrast:
[0091] Known distortion map X and natural image Y, use and Represents the brightness information of two images:
[0092]
[0093] where x i ,y i are the pixel values of the know...
PUM
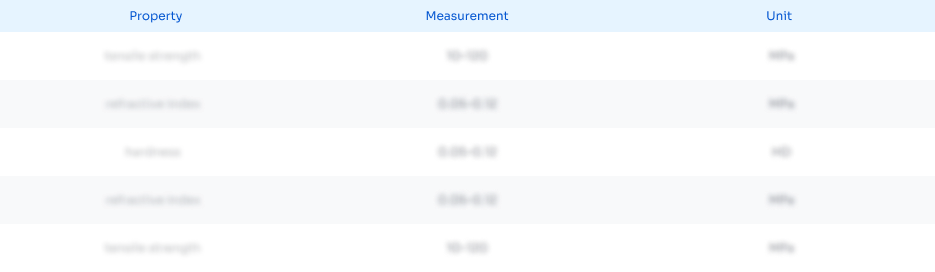
Abstract
Description
Claims
Application Information
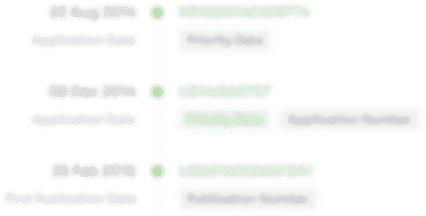
- R&D Engineer
- R&D Manager
- IP Professional
- Industry Leading Data Capabilities
- Powerful AI technology
- Patent DNA Extraction
Browse by: Latest US Patents, China's latest patents, Technical Efficacy Thesaurus, Application Domain, Technology Topic, Popular Technical Reports.
© 2024 PatSnap. All rights reserved.Legal|Privacy policy|Modern Slavery Act Transparency Statement|Sitemap|About US| Contact US: help@patsnap.com