Neural network acceleration method based on cross-resolution knowledge distillation
A neural network and resolution technology, applied in the field of deep learning, can solve problems such as unfavorable neural network applications, reduce the robustness and generalization ability of deep features, and do not consider the impact of the computational complexity of input images, and achieve low computational complexity. speed, increase the operation speed, and reduce the resolution
- Summary
- Abstract
- Description
- Claims
- Application Information
AI Technical Summary
Problems solved by technology
Method used
Image
Examples
Embodiment 1
[0042] Such as figure 1 As shown, this embodiment provides a neural network acceleration method based on cross-resolution knowledge distillation, which includes a high-resolution teacher network and a low-resolution student network, wherein the high-resolution teacher network is obtained from high-resolution training samples To learn and extract robust feature representations, the low-resolution student network quickly extracts deep features through low-resolution inputs, and extracts the prior knowledge of the high-resolution teacher network through a cross-resolution knowledge distillation loss to improve the discriminative ability of features.
[0043] In this embodiment, it is first necessary to obtain high-resolution training samples and low-resolution training samples by resampling according to the requirements of the application environment, and construct a high-resolution teacher network and a low-resolution student network, mainly including obtaining sample data, high-...
PUM
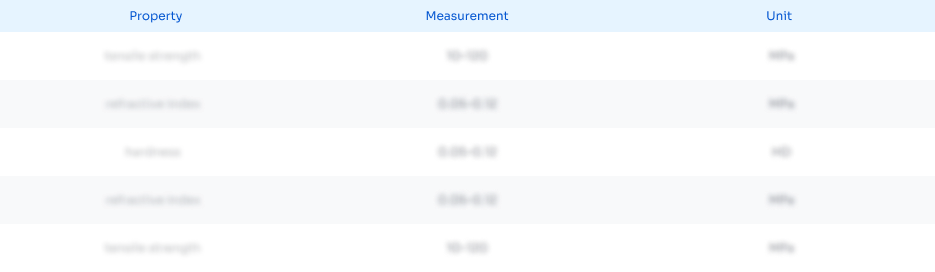
Abstract
Description
Claims
Application Information
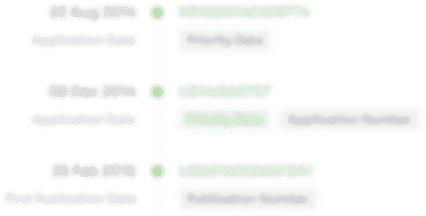
- R&D Engineer
- R&D Manager
- IP Professional
- Industry Leading Data Capabilities
- Powerful AI technology
- Patent DNA Extraction
Browse by: Latest US Patents, China's latest patents, Technical Efficacy Thesaurus, Application Domain, Technology Topic, Popular Technical Reports.
© 2024 PatSnap. All rights reserved.Legal|Privacy policy|Modern Slavery Act Transparency Statement|Sitemap|About US| Contact US: help@patsnap.com