Image super-resolution reconstruction method based on multi-scale attention cascade network
A technology of super-resolution reconstruction and cascade network, which is applied in the field of image super-resolution reconstruction based on multi-scale attention cascade network, can solve the problems of disappearing feature information, ignoring the full use of LR images, and unfavorable image detail learning, etc., to achieve The effect of intuitive feature extraction
- Summary
- Abstract
- Description
- Claims
- Application Information
AI Technical Summary
Problems solved by technology
Method used
Image
Examples
Embodiment Construction
[0048] The present invention provides an image super-resolution reconstruction method of a multi-scale attention cascaded network. The method first uses a convolution operation to extract shallow features of a low-resolution image; then, the shallow features are input into a feature extraction subnetwork, Obtain cascaded features; further, pass the cascaded features through a convolution layer with a convolution kernel of 1 to obtain an optimized feature vector; input the optimized feature vector into the image deep learning upsampling module to obtain a reconstructed image Meanwhile, for low-resolution images I LR Using interpolation algorithm to obtain reconstructed image Finally, the image will be reconstructed and fusion to obtain the final high-resolution reconstructed image I SR . The present invention is suitable for super-resolution reconstruction of images. Using the present invention for super-resolution reconstruction, the obtained high-resolution image has ...
PUM
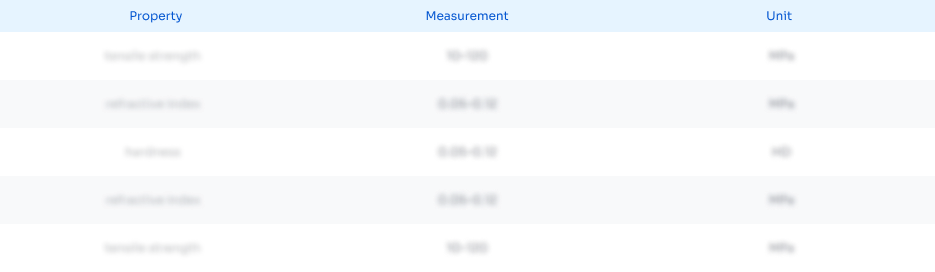
Abstract
Description
Claims
Application Information
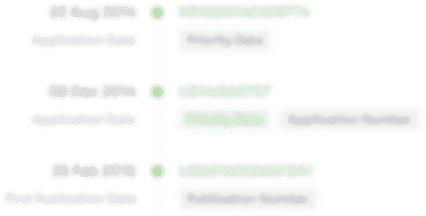
- R&D Engineer
- R&D Manager
- IP Professional
- Industry Leading Data Capabilities
- Powerful AI technology
- Patent DNA Extraction
Browse by: Latest US Patents, China's latest patents, Technical Efficacy Thesaurus, Application Domain, Technology Topic, Popular Technical Reports.
© 2024 PatSnap. All rights reserved.Legal|Privacy policy|Modern Slavery Act Transparency Statement|Sitemap|About US| Contact US: help@patsnap.com