Traffic flow prediction method of deep network based on fusion of spatiotemporal features
A space-time feature and deep network technology, applied in traffic flow detection, road vehicle traffic control system, traffic control system, etc., can solve problems such as incomplete feature fusion and incomplete feature extraction
- Summary
- Abstract
- Description
- Claims
- Application Information
AI Technical Summary
Problems solved by technology
Method used
Image
Examples
Embodiment Construction
[0082] The present invention will be further described below in conjunction with the accompanying drawings and embodiments.
[0083] Please refer to figure 1 , the present invention provides a traffic flow prediction method based on a deep network of fusion spatiotemporal features, comprising the following steps:
[0084] Step A: Obtain historical traffic flow data containing spatio-temporal information, and build a traffic flow data training set;
[0085] Step B: preprocessing the traffic flow data training set to obtain the spatio-temporal matrix representation of the historical traffic flow data;
[0086] Step C: Using the space-time matrix representation as the input of the deep learning network, train the deep learning network TSNN;
[0087] Step D: Input the traffic flow data sequence to be predicted into the trained deep learning network to obtain the prediction result.
[0088] In this embodiment, the step A is specifically:
[0089] Step A1: Obtain the monitoring ...
PUM
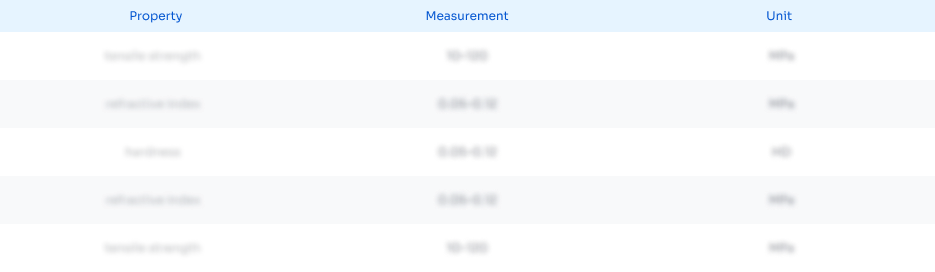
Abstract
Description
Claims
Application Information
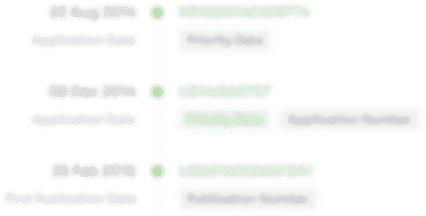
- R&D
- Intellectual Property
- Life Sciences
- Materials
- Tech Scout
- Unparalleled Data Quality
- Higher Quality Content
- 60% Fewer Hallucinations
Browse by: Latest US Patents, China's latest patents, Technical Efficacy Thesaurus, Application Domain, Technology Topic, Popular Technical Reports.
© 2025 PatSnap. All rights reserved.Legal|Privacy policy|Modern Slavery Act Transparency Statement|Sitemap|About US| Contact US: help@patsnap.com