Chest radiography multi-label classification method based on self-correcting label generation network
A classification method and multi-label technology, applied in the field of medical image processing, can solve problems such as unbalanced prediction effects, and achieve the effects of alleviating the unbalanced phenomenon of model prediction, improving performance, and improving prediction accuracy.
- Summary
- Abstract
- Description
- Claims
- Application Information
AI Technical Summary
Problems solved by technology
Method used
Image
Examples
Embodiment Construction
[0036] It can be seen from the background technology that most of the previous research regards multi-label as an independent single-label problem and ignores the correlation between labels. This ultimately leads to an imbalance in the model's prediction performance, that is, it performs well on easier-to-predict disease labels, but performs poorly on harder-to-predict disease labels, such as pneumonia.
[0037]The present invention conducts further research on the above problems. The self-modifying label generation network (SLGN) provided in the present invention can simultaneously capture and utilize the spatial association, semantic association and co-occurrence between labels, thereby maximizing the improvement of the model. prediction accuracy. The present invention employs an encoder-decoder structure. In image captioning tasks, CNNs are used as encoders to extract image features. Image features are an abstract representation of images, including high-level semantic in...
PUM
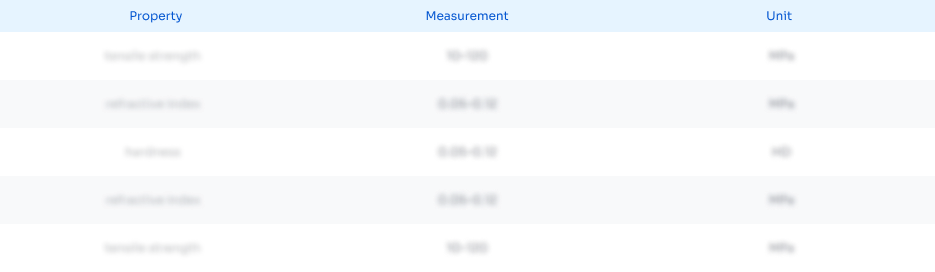
Abstract
Description
Claims
Application Information
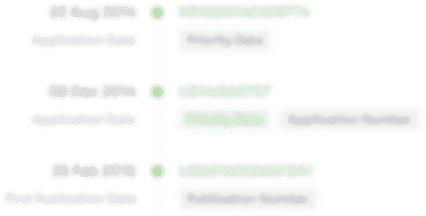
- Generate Ideas
- Intellectual Property
- Life Sciences
- Materials
- Tech Scout
- Unparalleled Data Quality
- Higher Quality Content
- 60% Fewer Hallucinations
Browse by: Latest US Patents, China's latest patents, Technical Efficacy Thesaurus, Application Domain, Technology Topic, Popular Technical Reports.
© 2025 PatSnap. All rights reserved.Legal|Privacy policy|Modern Slavery Act Transparency Statement|Sitemap|About US| Contact US: help@patsnap.com