Distributed machine learning method capable of tolerating untrusted nodes
A machine learning and distributed technology, applied in machine learning, integrated learning, instruments, etc., can solve problems such as weak working nodes, difficulty in ensuring the credibility of working nodes, untrustworthy working nodes, etc., and achieve the effect of improving robustness
- Summary
- Abstract
- Description
- Claims
- Application Information
AI Technical Summary
Problems solved by technology
Method used
Image
Examples
Embodiment Construction
[0034] Below in conjunction with specific embodiments, the present invention will be further illustrated, and it should be understood that these embodiments are only used to illustrate the present invention and not to limit the scope of the present invention. The modifications all fall within the scope defined by the appended claims of this application.
[0035] The distributed machine learning method that can tolerate untrusted nodes provided by the present invention can be applied not only to multi-machine cluster distributed machine learning, but also to edge computing, federated learning and other applications, and is suitable for a large number of data sets to be classified. , The scene where the machine learning model used has a large number of parameters is also suitable for the scene where the data is distributed on each terminal, but the training data cannot be sent due to various reasons. The present invention can be applied to various tasks such as image classificat...
PUM
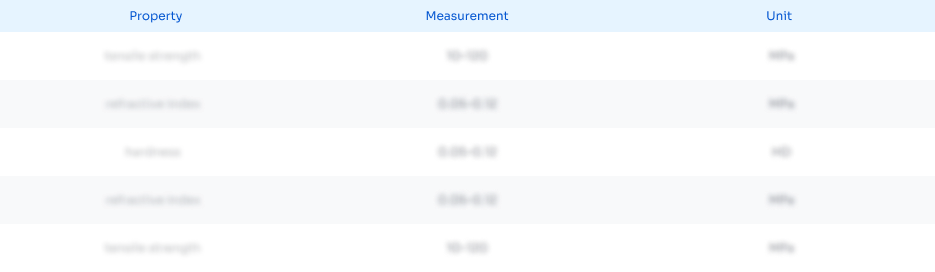
Abstract
Description
Claims
Application Information
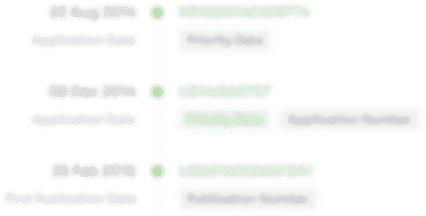
- Generate Ideas
- Intellectual Property
- Life Sciences
- Materials
- Tech Scout
- Unparalleled Data Quality
- Higher Quality Content
- 60% Fewer Hallucinations
Browse by: Latest US Patents, China's latest patents, Technical Efficacy Thesaurus, Application Domain, Technology Topic, Popular Technical Reports.
© 2025 PatSnap. All rights reserved.Legal|Privacy policy|Modern Slavery Act Transparency Statement|Sitemap|About US| Contact US: help@patsnap.com