Automobile automatic driving motion planning method and system based on learning sampling mode
A technology of motion planning and automatic driving, which is applied in control/regulation systems, motor vehicles, transportation and packaging, etc. It can solve problems such as difficult to guarantee solution time, difficult engineering practice and optimization, difficult to adapt to complex and changeable cost functions, etc. , to achieve the effect of reasonable trajectory selection, improved safety and robustness
- Summary
- Abstract
- Description
- Claims
- Application Information
AI Technical Summary
Problems solved by technology
Method used
Image
Examples
Embodiment Construction
[0024] The present invention will be described in detail below in conjunction with the accompanying drawings and embodiments.
[0025] Such as figure 1 As shown, the present invention provides a learning-sampling-based automatic driving motion planning method. This method combines reinforcement learning and receives automatic driving perception results, that is, information such as the position and speed of obstacles in the surrounding environment. Under the constraints of the location, output a series of actions that the intelligent vehicle can perform.
[0026] Specifically include the following steps:
[0027] S1: Establish vehicle kinematics model according to vehicle parameters;
[0028] Among them, the vehicle parameters include vehicle size, minimum turning radius, maximum acceleration\deceleration, etc.
[0029] S2: Initialize the storage tables of the heuristic motion planning method: Open table and Closed table.
[0030] S3: Based on the learning sampling method, a...
PUM
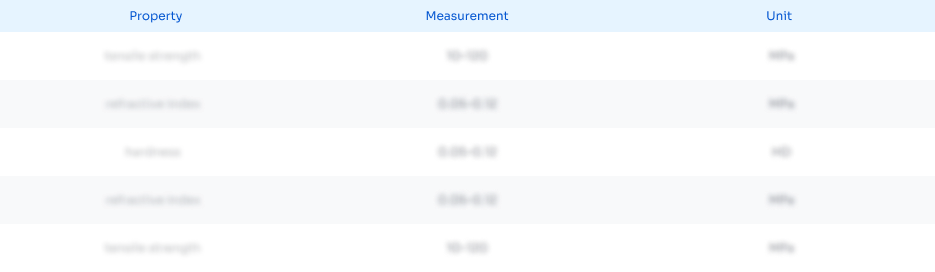
Abstract
Description
Claims
Application Information
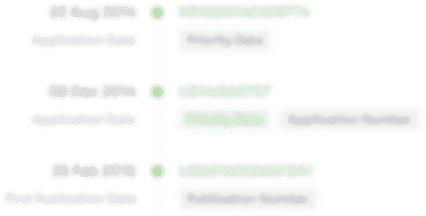
- R&D Engineer
- R&D Manager
- IP Professional
- Industry Leading Data Capabilities
- Powerful AI technology
- Patent DNA Extraction
Browse by: Latest US Patents, China's latest patents, Technical Efficacy Thesaurus, Application Domain, Technology Topic, Popular Technical Reports.
© 2024 PatSnap. All rights reserved.Legal|Privacy policy|Modern Slavery Act Transparency Statement|Sitemap|About US| Contact US: help@patsnap.com