Multi-label text data feature selection method and device
A technology of data features and text data, applied in the fields of electrical digital data processing, natural language data processing, instruments, etc., can solve problems such as low accuracy and complex algorithms
- Summary
- Abstract
- Description
- Claims
- Application Information
AI Technical Summary
Problems solved by technology
Method used
Image
Examples
Embodiment Construction
[0046] The specific embodiments of the present invention will be further described below in conjunction with the accompanying drawings.
[0047] method embodiment
[0048] Before introducing the specific means of the present invention, some knowledge related to the present invention, the Fisher-Score algorithm and the neighborhood rough set algorithm are introduced.
[0049] 1) Related concepts of mutual information
[0050] Assuming that A and B are two events, and P(A)>0, the conditional probability of event B under the condition that event A occurs is:
[0051]
[0052] For a discrete random variable X={x 1 ,x 2 ,...,x n}, then the information entropy of random variable X can be expressed as:
[0053]
[0054] In the formula, P(x i ) is the occurrence of event x i The probability of; n is the total number of possible events (states). Obviously, for a fully determined variable X, H(X)=0; for a random variable X, H(X)>0 (non-negativity), and the value of H(X) in...
PUM
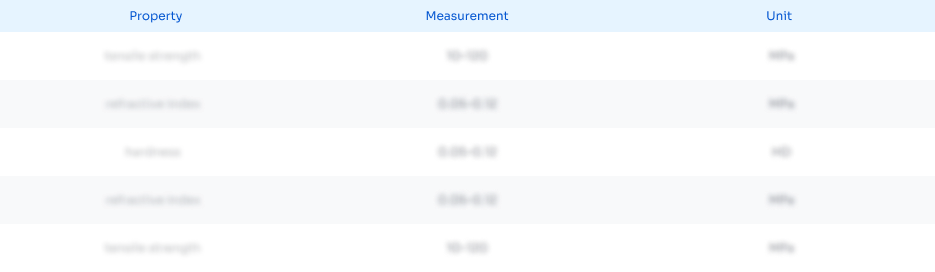
Abstract
Description
Claims
Application Information
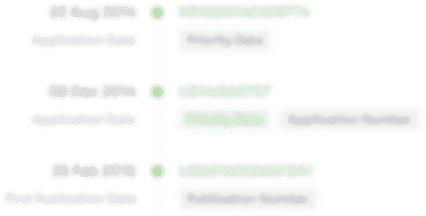
- R&D Engineer
- R&D Manager
- IP Professional
- Industry Leading Data Capabilities
- Powerful AI technology
- Patent DNA Extraction
Browse by: Latest US Patents, China's latest patents, Technical Efficacy Thesaurus, Application Domain, Technology Topic, Popular Technical Reports.
© 2024 PatSnap. All rights reserved.Legal|Privacy policy|Modern Slavery Act Transparency Statement|Sitemap|About US| Contact US: help@patsnap.com