Accurate facial paralysis degree evaluation method and device based on face feature points
An evaluation method and face feature technology, applied in neural learning methods, instruments, biological neural network models, etc., can solve problems such as large errors and low evaluation efficiency, and achieve high accuracy, high evaluation efficiency, and high detection and positioning accuracy Effect
- Summary
- Abstract
- Description
- Claims
- Application Information
AI Technical Summary
Problems solved by technology
Method used
Image
Examples
Embodiment 1
[0069] see figure 1 , the present embodiment provides a method for accurately evaluating the degree of facial paralysis based on facial feature points, which can be applied to facial paralysis detection equipment as a detection method for medical equipment to detect the degree of facial paralysis of patients with facial paralysis, and can be large-scale, Extensive industrial application, for example, it can be used as an independent program in the mobile terminal and client terminal, which can be used for correction and inspection of patients with facial paralysis during the non-treatment period, and can also be used as a preventive method for patients without facial paralysis. Wherein, the accurate facial paralysis evaluation method includes the following steps.
[0070] Step (1): Establish a facial paralysis key point detection model. In this embodiment, the method for establishing the facial paralysis key point detection model includes the following steps, namely steps (1....
Embodiment 2
[0092] see image 3 , this embodiment provides a method for accurately evaluating the degree of facial paralysis based on facial feature points, which is similar to that of Embodiment 1, except that the depth fully convolutional network model of this embodiment is different. The specific structure of the deep full convolutional network model in this embodiment can be designed separately according to the specific requirements of users. For the convenience of further introduction, an example of the structure of a deep full convolutional network model is now designed. image 3 shown. The number of downsampling and upsampling layers of the deep full convolutional network model is 3 layers, and the downsampling adopts maxpooling maximum pooling method. The size of the pooling layer is 2×2 and the step size is 2. The upsampling adopts The dconv deconvolution method, the size of the deconvolution layer is 2×2 and the step size is 2. Each adjacent upsampling or downsampling is separa...
Embodiment 3
[0094] This embodiment provides a device for evaluating the degree of accurate facial paralysis based on facial feature points, which uses the method for evaluating the degree of accurate facial paralysis based on facial feature points in Embodiment 1 or Embodiment 2. Among them, the precise facial paralysis degree evaluation device includes a detection model building module, a data acquisition module, a data processing module and a facial paralysis comprehensive evaluation module. The data acquisition module and the data processing module can form a data acquisition and processing module to be detected. These modules can be used as computer program modules or hardware modules, which can execute the relevant steps described in Embodiment 1 or Embodiment 2.
[0095] The detection model building module is used to set up a facial paralysis key point detection model, which is actually used to implement step (1) in Embodiment 1. In the facial paralysis key point detection model, de...
PUM
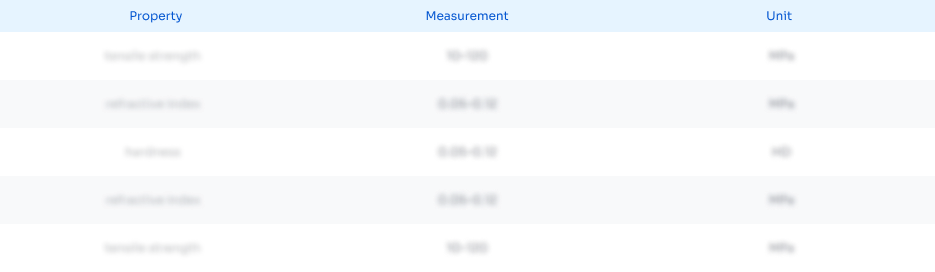
Abstract
Description
Claims
Application Information
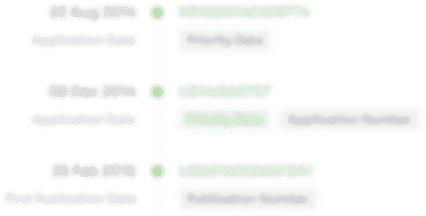
- R&D Engineer
- R&D Manager
- IP Professional
- Industry Leading Data Capabilities
- Powerful AI technology
- Patent DNA Extraction
Browse by: Latest US Patents, China's latest patents, Technical Efficacy Thesaurus, Application Domain, Technology Topic.
© 2024 PatSnap. All rights reserved.Legal|Privacy policy|Modern Slavery Act Transparency Statement|Sitemap