Tunnel surface defect detection method based on convolutional neural network
A convolutional neural network and defect detection technology, applied in the field of image processing and analysis, can solve the problems of high operating cost, low classification accuracy, and low detection speed of the magnetic particle method, so as to eliminate the interference of human subjective factors, save labor costs, The effect of high accuracy
- Summary
- Abstract
- Description
- Claims
- Application Information
AI Technical Summary
Problems solved by technology
Method used
Image
Examples
Embodiment Construction
[0026] In order to further clarify the working principle and working process of the present invention, the method of the present invention will be described in detail below in conjunction with the accompanying drawings and specific embodiments.
[0027] Such as figure 1 As shown, a method for detecting surface defects of a tunnel based on a convolutional neural network of the present invention specifically includes the following steps:
[0028] S1: Obtain tunnel image data; such as figure 2 As shown in Fig. 1, the image data of the tunnel surface to be tested is obtained by using an industrial camera. The image collection process is comprehensive, including sample pictures of different areas of the tunnel, as well as pictures with defects and pictures without defects. The surface defects of the tunnel include: water seepage, cracks, shedding, defects, etc.
[0029] S2: if image 3 As shown, the acquired image data is preprocessed, and the data set is divided into a test da...
PUM
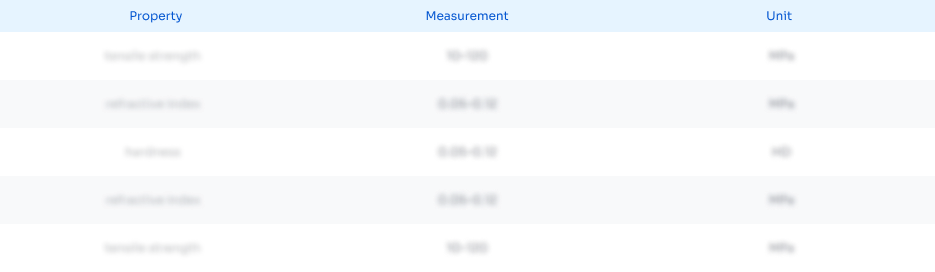
Abstract
Description
Claims
Application Information
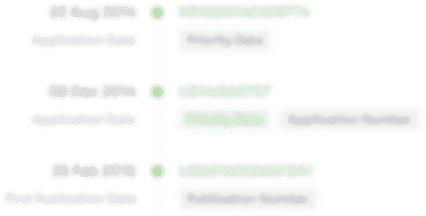
- R&D Engineer
- R&D Manager
- IP Professional
- Industry Leading Data Capabilities
- Powerful AI technology
- Patent DNA Extraction
Browse by: Latest US Patents, China's latest patents, Technical Efficacy Thesaurus, Application Domain, Technology Topic.
© 2024 PatSnap. All rights reserved.Legal|Privacy policy|Modern Slavery Act Transparency Statement|Sitemap