Printed matter defect identification method based on deep convolution generative adversarial network
A technology of deep convolution and defect recognition, which is applied in character and pattern recognition, biological neural network models, neural learning methods, etc., can solve the problems of reduced recognition rate, large amount of data, difficult data, etc., to increase the amount of training samples, improve Detection efficiency and accuracy, the effect of slow resolution
- Summary
- Abstract
- Description
- Claims
- Application Information
AI Technical Summary
Problems solved by technology
Method used
Image
Examples
Embodiment 1
[0033] Such as figure 1 As shown, a method for identifying defects in printed matter based on deep convolutional generative confrontation network, which includes the following steps:
[0034] Step 1, collecting pictures of printed matter to be recognized, and using the picture of printed matter to be recognized as a training sample.
[0035] Step 2, construct a deep convolutional generative confrontation network.
[0036] Step 3: Input the image of printed matter to be identified into the deep convolutional generative adversarial network to generate image samples of printed matter.
[0037] Step 4, using the image of the printed matter to be identified and the generated image samples of the printed matter to generate a training sample set.
[0038] Step 5, construct a convolutional neural network and use the training sample set to train the convolutional neural network.
[0039] Step 6, using the trained convolutional neural network to detect print defects.
[0040] As a p...
PUM
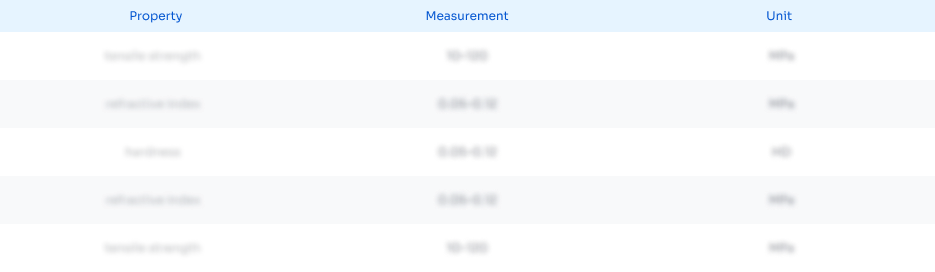
Abstract
Description
Claims
Application Information
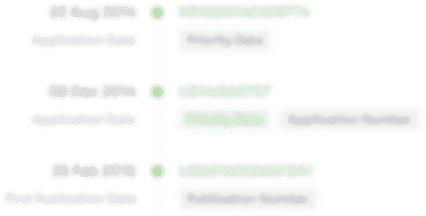
- R&D Engineer
- R&D Manager
- IP Professional
- Industry Leading Data Capabilities
- Powerful AI technology
- Patent DNA Extraction
Browse by: Latest US Patents, China's latest patents, Technical Efficacy Thesaurus, Application Domain, Technology Topic, Popular Technical Reports.
© 2024 PatSnap. All rights reserved.Legal|Privacy policy|Modern Slavery Act Transparency Statement|Sitemap|About US| Contact US: help@patsnap.com