Method for resisting attack deep neural network based on frequency band
A deep neural network and frequency band technology, which is applied in the field of adversarial attack deep neural network based on frequency band, can solve the problems of difficulty in explaining the law of anti-interference, and achieve the effect of improving sensitivity and reducing the intensity of interference.
- Summary
- Abstract
- Description
- Claims
- Application Information
AI Technical Summary
Problems solved by technology
Method used
Image
Examples
Embodiment
[0059] This embodiment provides a method for countering attacking deep neural networks based on frequency bands, comprising the following steps:
[0060] Step 1. Set the anti-interference strength, which is limited by the L∞ norm eps; when the anti-interference strength is limited by the L∞ norm eps, it is difficult for people to detect it; under the same interference strength, the attack depth neural During the network, a fair comparison has been realized; according to the anti-jamming strength set, the number of directions N of the frequency band and the number of wavelengths M of the frequency band are determined; wherein, in the present embodiment, N=8, M=5; half wavelength = {1, 2, 3, 4, 5};
[0061] Step 2. For the frequency band anti-interference distribution of a certain wavelength, calculate the frequency band anti-interference distribution in the horizontal direction; specifically, first initialize the frequency band anti-interference distribution in the horizontal d...
PUM
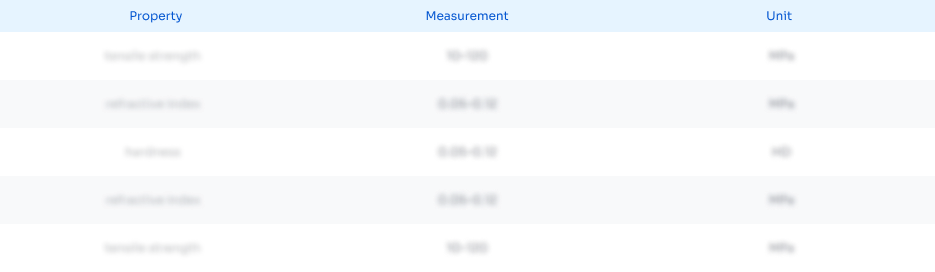
Abstract
Description
Claims
Application Information
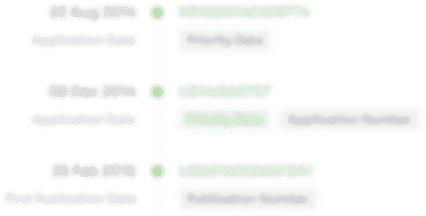
- R&D
- Intellectual Property
- Life Sciences
- Materials
- Tech Scout
- Unparalleled Data Quality
- Higher Quality Content
- 60% Fewer Hallucinations
Browse by: Latest US Patents, China's latest patents, Technical Efficacy Thesaurus, Application Domain, Technology Topic, Popular Technical Reports.
© 2025 PatSnap. All rights reserved.Legal|Privacy policy|Modern Slavery Act Transparency Statement|Sitemap|About US| Contact US: help@patsnap.com