Image processing method and system, electronic equipment and storage medium
A technology in image processing and image, applied in the field of image processing, can solve the problems of quantization precision loss, quantization sawtooth, quantization ripple, etc., and achieve the effect of reducing delay, saving bandwidth, and reducing power consumption
- Summary
- Abstract
- Description
- Claims
- Application Information
AI Technical Summary
Problems solved by technology
Method used
Image
Examples
Embodiment 1
[0058] This embodiment provides an image processing method, such as figure 1 shown, including:
[0059] Step S101. Input the RGB values of all pixels in the image to the integer-quantized deep neural network model; wherein, the output and weight of each layer of the deep neural network model are quantized as integers.
[0060] Wherein, the above-mentioned image may be a static picture, or a video frame in a dynamic video. It should be noted that the RGB value of a pixel is an integer type int. In one example, the RGB value of a certain pixel is (220, 54, 255). In the specific implementation of step S101, the RGB values of all pixels in the image are input into the integer-quantized deep neural network model in the form of a matrix.
[0061] In an alternative embodiment, such as figure 2 As shown, the deep neural network model specifically includes a feature extraction module, an ASPP module, a decoding module and a portrait matting module.
[0062] The feature extract...
Embodiment 2
[0094] This embodiment provides an image processing system 300, such as image 3 As shown, an input unit 301 and an inverse quantization unit 302 are included.
[0095] The input unit 301 is used to input the RGB values of all pixels in the image to the integer-quantized deep neural network model; wherein, the output and weight of each layer of the deep neural network model are quantized as integers.
[0096] The dequantization unit 302 is used to dequantize the integer probability array output by the deep neural network model into a floating-point probability array, so as to realize portrait matting, wherein the probability array includes each pixel in the image The point is the probability of the foreground portrait.
[0097] In an optional implementation manner, the image processing system further includes a fusion unit, configured to fuse the foreground portrait separated from the image with the preset background picture according to the floating-point probability array...
Embodiment 3
[0112] Figure 4 A schematic structural diagram of an electronic device provided in this embodiment. The electronic device includes a memory, a processor, and a computer program stored on the memory and operable on the processor, and the processor implements the image processing method of Embodiment 1 when executing the program. Figure 4 The electronic device 3 shown is only an example, and should not impose any limitation on the functions and application scope of the embodiments of the present invention.
[0113] The electronic device 3 may be in the form of a general computing device, eg it may be a server device. Components of the electronic device 3 may include but not limited to: the at least one processor 4 mentioned above, the at least one memory 5 mentioned above, and the bus 6 connecting different system components (including the memory 5 and the processor 4 ).
[0114] The bus 6 includes a data bus, an address bus and a control bus.
[0115] The memory 5 may incl...
PUM
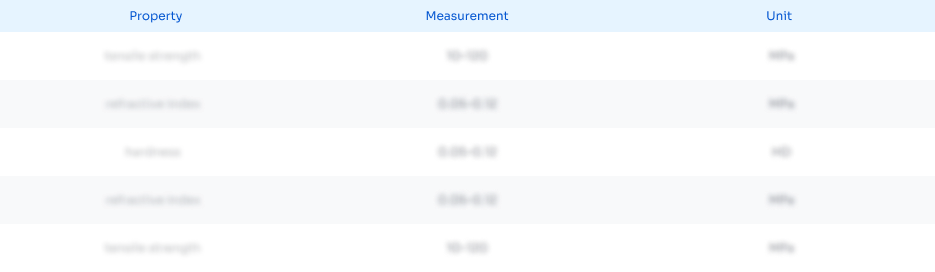
Abstract
Description
Claims
Application Information
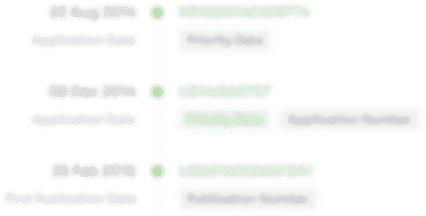
- R&D Engineer
- R&D Manager
- IP Professional
- Industry Leading Data Capabilities
- Powerful AI technology
- Patent DNA Extraction
Browse by: Latest US Patents, China's latest patents, Technical Efficacy Thesaurus, Application Domain, Technology Topic.
© 2024 PatSnap. All rights reserved.Legal|Privacy policy|Modern Slavery Act Transparency Statement|Sitemap