Remote equipment health prediction method based on machine learning and edge computing
A technology of edge computing and remote equipment, applied in the direction of instruments, electrical testing/monitoring, testing/monitoring control systems, etc., can solve problems such as reduced management efficiency, impact on production activities, property loss, etc., to achieve the effect of reducing impact
- Summary
- Abstract
- Description
- Claims
- Application Information
AI Technical Summary
Problems solved by technology
Method used
Image
Examples
Example Embodiment
[0026] The preferred embodiments of the present invention will be described below in conjunction with the accompanying drawings. It should be understood that the preferred embodiments described here are only used to illustrate and explain the present invention, and are not used to limit the present invention.
[0027] Please refer to Figure 1-2 As shown, a remote device health prediction method based on machine learning and edge computing. This method deploys edge computing terminals that integrate computing, storage, network, and application core capabilities on the edge side close to the device or data source. Edge computing terminals Combine the communication module to realize the connection with the production equipment, and collect the operation element data of the production equipment in real time. The edge computing terminal includes an edge gateway, a network, a cloud data platform and an application terminal; the specific prediction steps are:
[0028] S1: Collect real-ti...
PUM
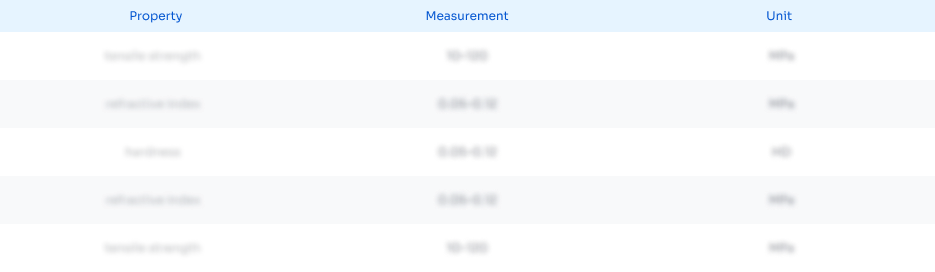
Abstract
Description
Claims
Application Information
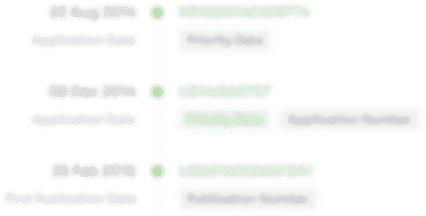
- R&D Engineer
- R&D Manager
- IP Professional
- Industry Leading Data Capabilities
- Powerful AI technology
- Patent DNA Extraction
Browse by: Latest US Patents, China's latest patents, Technical Efficacy Thesaurus, Application Domain, Technology Topic.
© 2024 PatSnap. All rights reserved.Legal|Privacy policy|Modern Slavery Act Transparency Statement|Sitemap