Single-image robot disordered target grabbing method based on pose estimation and correction
A pose estimation and single-image technology, applied in the field of intelligent robots, can solve problems such as excessive power consumption, difficulty in detection, and applicability dependence
- Summary
- Abstract
- Description
- Claims
- Application Information
AI Technical Summary
Problems solved by technology
Method used
Image
Examples
Embodiment Construction
[0107] In order to enable those skilled in the art to better understand the technical solutions of the present invention, the present invention will be further described in detail below in conjunction with the accompanying drawings.
[0108] Such as figure 1 Shown, a single-image robot disordered target grasping method based on pose estimation and correction, the method includes the following steps:
[0109] S1. Obtain random image data and realistic image data of the object model to be captured, and generate a corresponding image data set;
[0110] S2. Constructing a convolutional neural network, and inputting the image dataset acquired in step S1 into the convolutional neural network for off-line training to obtain a convolutional neural network model;
[0111] S3. Collecting the two-dimensional image of the object to be grasped through the depth camera and importing the two-dimensional image into the convolutional neural network model, and outputting the corresponding conf...
PUM
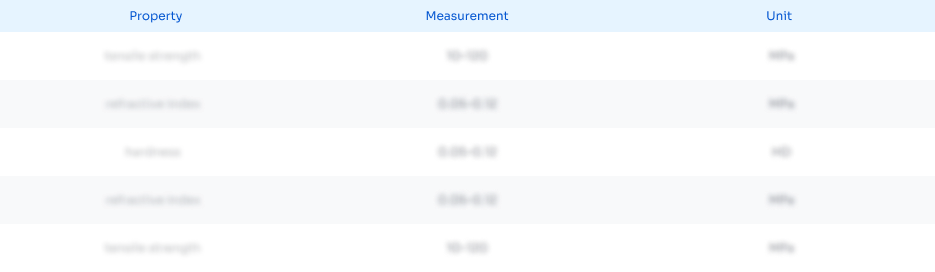
Abstract
Description
Claims
Application Information
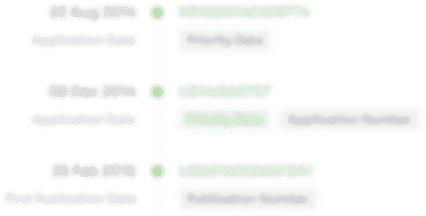
- R&D Engineer
- R&D Manager
- IP Professional
- Industry Leading Data Capabilities
- Powerful AI technology
- Patent DNA Extraction
Browse by: Latest US Patents, China's latest patents, Technical Efficacy Thesaurus, Application Domain, Technology Topic, Popular Technical Reports.
© 2024 PatSnap. All rights reserved.Legal|Privacy policy|Modern Slavery Act Transparency Statement|Sitemap|About US| Contact US: help@patsnap.com