Air quality space-time prediction method based on long-term and short-term memory neural network
A long-term and short-term memory, air quality technology, applied in neural learning methods, biological neural network models, prediction and other directions, can solve problems such as interference model accuracy, ignoring interaction, influence, etc.
- Summary
- Abstract
- Description
- Claims
- Application Information
AI Technical Summary
Problems solved by technology
Method used
Image
Examples
Embodiment Construction
[0033] The present invention will be further described below in conjunction with accompanying drawing.
[0034] Such as figure 1 with 2 Shown, the air quality spatio-temporal prediction method based on long short-term memory neural network of the present invention, concrete steps are as follows:
[0035] Step 1: Obtain historical air quality data and meteorological data;
[0036] Step 2: Perform data preprocessing on historical air quality, including outlier elimination, missing value interpolation, extraction of particle concentration data at adjacent stations, and data normalization;
[0037] Step 3: Convert the data format and divide the data set. From sequences to pairs of input and output sequences; split the dataset into training and testing sets;
[0038] Step 4: Initialize various parameters of the long-term short-term memory network (LSTM), and input the data of the training set into the long-term short-term memory neural network for training until the network con...
PUM
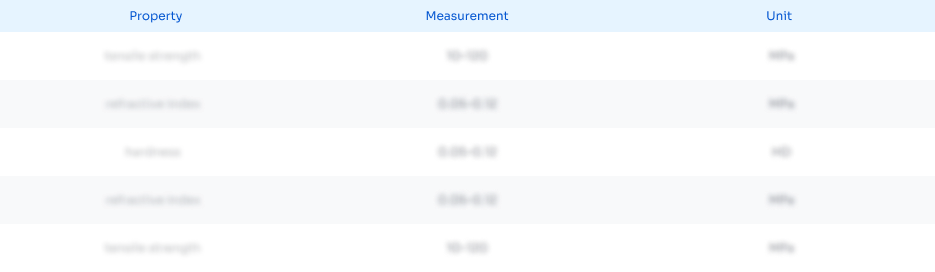
Abstract
Description
Claims
Application Information
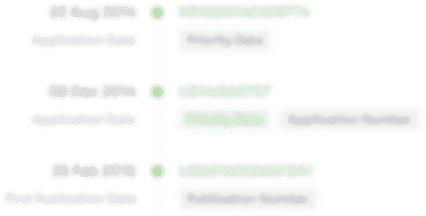
- R&D
- Intellectual Property
- Life Sciences
- Materials
- Tech Scout
- Unparalleled Data Quality
- Higher Quality Content
- 60% Fewer Hallucinations
Browse by: Latest US Patents, China's latest patents, Technical Efficacy Thesaurus, Application Domain, Technology Topic, Popular Technical Reports.
© 2025 PatSnap. All rights reserved.Legal|Privacy policy|Modern Slavery Act Transparency Statement|Sitemap|About US| Contact US: help@patsnap.com