Adaptive feature and data distribution target detection method
A technology for target detection and data distribution, which is applied in the field of computer vision and can solve the problems of parameter redundancy, uncertainty, and concentrated target size distribution.
- Summary
- Abstract
- Description
- Claims
- Application Information
AI Technical Summary
Problems solved by technology
Method used
Image
Examples
Embodiment Construction
[0045] The object detection method of the self-adaptive feature and data distribution of the present invention uses the characteristic of feature multiplexing of DenseNet, and the last feature layer of DenseNet contains the features of all the previous channels with the same width and height. The present invention uses the idea of Inception structure to modify DenseNet, learns a weight for each channel of the last feature layer, multiplies each channel parameter by the weight, then directly discards the channel with lower weight, and finally sends it to the multi-FPN target Detection module to achieve the effect of automatically selecting the optimal depth feature for target detection. The specific method steps are:
[0046] 1) The input image adopts a width w of 416 pixels, a height h of 416 pixels, and the number of channels c of 3. The size of the image needs to be converted to 416x416 first. If the size of the input image is not 416x416, the input and output in the subs...
PUM
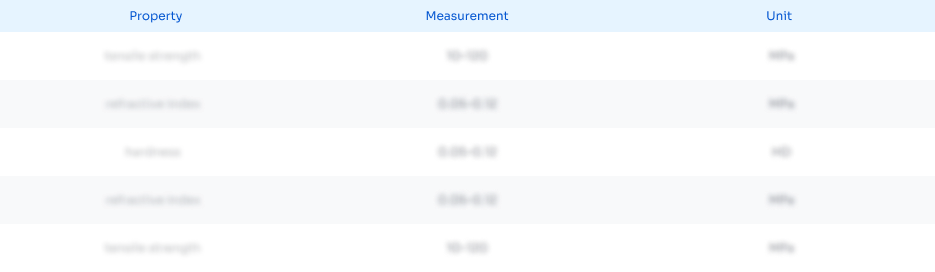
Abstract
Description
Claims
Application Information
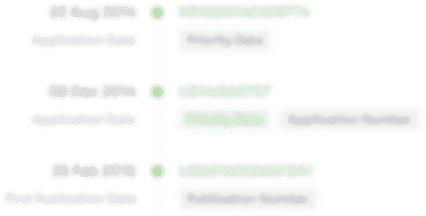
- R&D Engineer
- R&D Manager
- IP Professional
- Industry Leading Data Capabilities
- Powerful AI technology
- Patent DNA Extraction
Browse by: Latest US Patents, China's latest patents, Technical Efficacy Thesaurus, Application Domain, Technology Topic.
© 2024 PatSnap. All rights reserved.Legal|Privacy policy|Modern Slavery Act Transparency Statement|Sitemap