Flame target detection method based on digital image and convolution features
A target detection and digital image technology, applied in neural learning methods, character and pattern recognition, instruments, etc., can solve the problems of negligible effect of transfer learning and small object similarity, achieve high flexibility, high detection accuracy, reduce The effect of network parameters
- Summary
- Abstract
- Description
- Claims
- Application Information
AI Technical Summary
Problems solved by technology
Method used
Image
Examples
Embodiment Construction
[0034] The flame target detection method based on digital images and convolution features of the present invention will be described in detail below with examples.
[0035] 1 Dataset production
[0036] 1.1 Training set format
[0037] The model detects flames by extracting static features and dynamic features. Static features include features extracted by convolutional network and LBP texture features. Dynamic features include flame area change features, shape similarity features and flicker frequency features.
[0038]Static features must be extracted in real time according to the candidate frame during the training process, while dynamic features are extracted through video frames and have nothing to do with network parameter changes. Therefore, in order to facilitate model training and reduce redundant calculations, before model training, from the data set The extracted dynamic features are used together with the labeled images as the training set. Model training require...
PUM
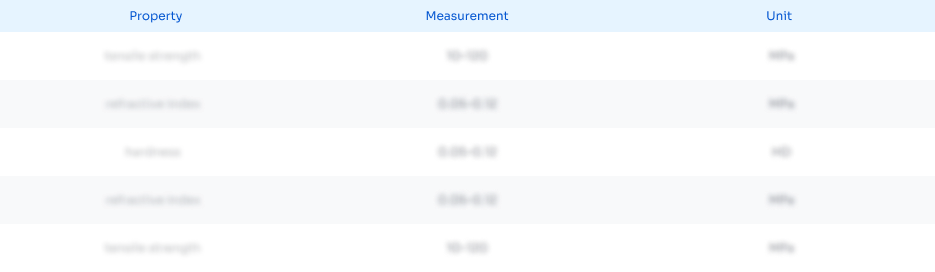
Abstract
Description
Claims
Application Information
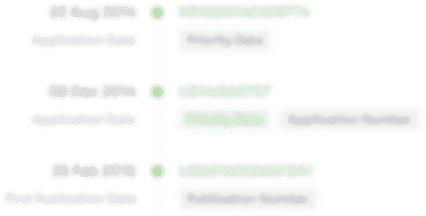
- R&D Engineer
- R&D Manager
- IP Professional
- Industry Leading Data Capabilities
- Powerful AI technology
- Patent DNA Extraction
Browse by: Latest US Patents, China's latest patents, Technical Efficacy Thesaurus, Application Domain, Technology Topic, Popular Technical Reports.
© 2024 PatSnap. All rights reserved.Legal|Privacy policy|Modern Slavery Act Transparency Statement|Sitemap|About US| Contact US: help@patsnap.com