Aeroelastic system flutter signal abnormal data expansion method
A technology of aeroelasticity and data expansion, applied in neural learning methods, biological neural network models, sustainable transportation, etc. effect of influence
- Summary
- Abstract
- Description
- Claims
- Application Information
AI Technical Summary
Problems solved by technology
Method used
Image
Examples
Embodiment Construction
[0031] Now in conjunction with embodiment, accompanying drawing, the present invention will be further described:
[0032] The implementation is divided into two parts, including the parameter optimization of the generative adversarial network and the independent application of the generator network.
[0033] First, according to the training and deployment principles of the deep learning algorithm, a data set is established for the designed network model, and the model is optimized and its parameters are optimized. The process is implemented based on the Pytorch deep learning framework.
[0034] In addition, since the GAN consists of two networks, one of which is the generator network and the other is the discriminator network, since this patent is mainly aimed at the data expansion of the chatter signal, therefore, based on the GAN to realize the chatter test signal Expansion. At this stage, the present invention is mainly aimed at data expansion in the subsequent data proce...
PUM
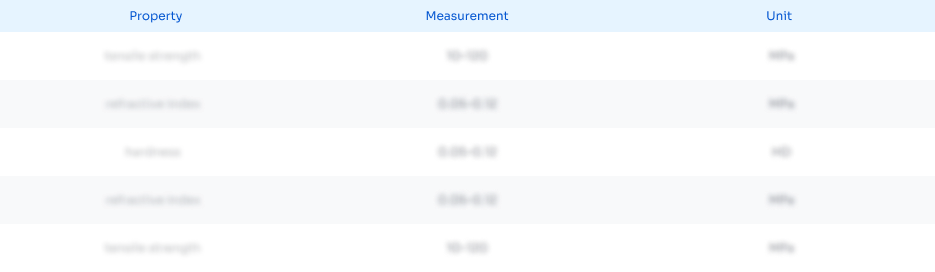
Abstract
Description
Claims
Application Information
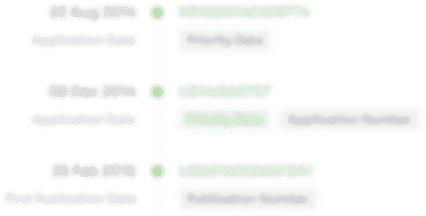
- R&D Engineer
- R&D Manager
- IP Professional
- Industry Leading Data Capabilities
- Powerful AI technology
- Patent DNA Extraction
Browse by: Latest US Patents, China's latest patents, Technical Efficacy Thesaurus, Application Domain, Technology Topic, Popular Technical Reports.
© 2024 PatSnap. All rights reserved.Legal|Privacy policy|Modern Slavery Act Transparency Statement|Sitemap|About US| Contact US: help@patsnap.com