Violent video recognition method for bimodal task learning based on attention mechanism
A video recognition and attention technology, applied in the field of violent video recognition, can solve the problems affecting the generalization ability of classifiers, ignoring interdependence, etc., to achieve the effect of improving generalization ability, improving feature appearance, and suppressing feature expression
- Summary
- Abstract
- Description
- Claims
- Application Information
AI Technical Summary
Problems solved by technology
Method used
Image
Examples
Embodiment 1
[0024] Embodiment 1: as figure 1 , figure 2 , image 3 and Figure 4 As shown, the violent video recognition method based on the dual-modal task learning of the attention mechanism includes the following steps:
[0025] Step 1: Add an attention mechanism module to the spatial flow deep neural network to capture the interdependence between the violent features of static frame pictures, and form the weight of the attention mechanism;
[0026] Step 2: Add an attention mechanism module to the time flow deep neural network to capture the interdependence between the violent features of the optical flow sequence diagram, and form the weight of the attention mechanism;
[0027] Step 3: Extract the feature information of the violent video on a single frame image, and establish a violent video recognition model based on a single frame image;
[0028] Step 4: Extract the feature information of the violent video on the motion optical flow, and establish a violent video recognition mo...
Embodiment 2
[0059] Embodiment 2: as figure 1 , figure 2 , image 3 and Figure 4 As shown, the violent video recognition method based on the dual-modal task learning of the attention mechanism includes the following steps:
[0060] Step S101, adding an attention mechanism module to the deep neural network to capture interdependence between violent features;
[0061] Step S102, using a deep neural network with an attention mechanism to extract the features of the violent video on a single frame image;
[0062] Step S103, using a deep neural network with an attention mechanism to extract the features of the violent video on the motion optical flow;
[0063] Step S104, build a more reasonable violence recognition system based on the post-fusion multi-feature average fusion strategy.
[0064] The first basic neural convolutional network used is the TSN network, which is composed of a spatial stream convolutional neural network and a temporal stream convolutional neural network. The atte...
PUM
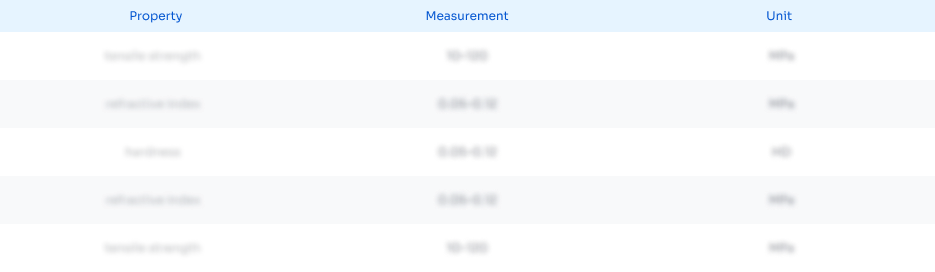
Abstract
Description
Claims
Application Information
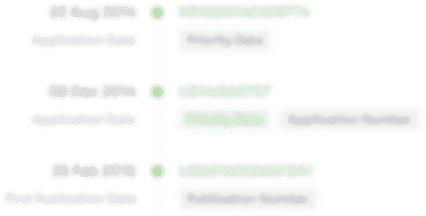
- R&D
- Intellectual Property
- Life Sciences
- Materials
- Tech Scout
- Unparalleled Data Quality
- Higher Quality Content
- 60% Fewer Hallucinations
Browse by: Latest US Patents, China's latest patents, Technical Efficacy Thesaurus, Application Domain, Technology Topic, Popular Technical Reports.
© 2025 PatSnap. All rights reserved.Legal|Privacy policy|Modern Slavery Act Transparency Statement|Sitemap|About US| Contact US: help@patsnap.com