Alzheimer's disease classification and prediction system based on multi-task learning
A multi-task learning and prediction system technology, applied in the field of Alzheimer's disease classification and prediction system, can solve the problem of not being able to judge whether individuals with mild cognitive impairment will transform into Alzheimer's disease, and achieve improved classification efficiency and accuracy, avoiding processing, and reducing the need for prior knowledge
- Summary
- Abstract
- Description
- Claims
- Application Information
AI Technical Summary
Problems solved by technology
Method used
Image
Examples
specific Embodiment approach 1
[0033] Specific implementation mode 1: In this implementation mode, a multi-task learning-based Alzheimer's disease classification and prediction system includes:
[0034] A multi-task learning-based Alzheimer's disease classification and prediction system according to the present invention is realized through the following technical solutions, including:
[0035] Structural magnetic resonance imaging (sMRI) preprocessing to reduce the effects of bias, noise and intensity in raw T1-weighted images and to unify image specifications;
[0036] Clinical indicator selection and feature extraction, that is, selecting clinical indicators for preprocessing and extracting feature vectors;
[0037] Disease classification and prediction model construction, that is, building each functional neural network module, adjusting the parameters of each module and building an overall neural network model, using the training sample set to train the disease classification and prediction model; usin...
specific Embodiment approach 2
[0047] Specific embodiment 2: The difference between this embodiment and specific embodiment 1 is that the image processing main module is used to collect head images, preprocess the collected head images, obtain preprocessed images, and The processed image is input into the training main module and the detection main module; the specific process is:
[0048] Obtain high-quality T1-weighted structural magnetic resonance imaging of the subject's head at the baseline time point (the time of the first visit, called the baseline time point) (Baseline) (high quality means that the patient's head position in the picture has no obvious shift , and the picture has no obvious artifacts; T1 weighting is a form of MRI image imaging);
[0049] Using the SPM tool (Statistical Parametric Mapping Toolbox) to perform skull stripping on structural magnetic resonance imaging;
[0050] Use non-rigid registration algorithms, including affine transformation and nonlinear transformation, etc., to ...
specific Embodiment approach 3
[0055] Embodiment 3: The difference between this embodiment and Embodiment 1 or 2 is that the clinical index processing main module is used to select the clinical index, obtain the feature vector of the clinical index, and input the feature vector of the clinical index into the training main module. module and detection main module; and the specific process is:
[0056] 1), selected clinical indicators;
[0057] 2), preprocessing the selected clinical indicators;
[0058] 3) Constructing a long-short-term memory neural network autoencoder (LSTM autoencoder) to extract the characteristics of longitudinal data of neuropsychological tests;
[0059] The clinical data includes baseline data and longitudinal data, and the features of the normalized baseline data and neuropsychological test longitudinal data are used as the input of Alzheimer's disease classification and prediction models.
[0060] Clinical indicators mainly include demographic information, genetic information and ...
PUM
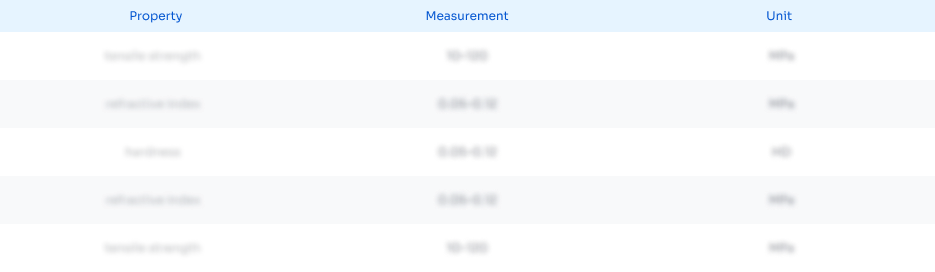
Abstract
Description
Claims
Application Information
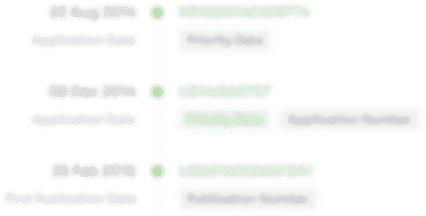
- R&D Engineer
- R&D Manager
- IP Professional
- Industry Leading Data Capabilities
- Powerful AI technology
- Patent DNA Extraction
Browse by: Latest US Patents, China's latest patents, Technical Efficacy Thesaurus, Application Domain, Technology Topic.
© 2024 PatSnap. All rights reserved.Legal|Privacy policy|Modern Slavery Act Transparency Statement|Sitemap