Weak and small human body target detection method based on accurate scale matching
A technology for human targets and detection methods, applied in the fields of computer vision and machine learning, can solve the problems of SM algorithm approximate processing uncertainty, imprecision, scale mismatch, etc., to balance information loss, improve performance, and promote similarity Effect
- Summary
- Abstract
- Description
- Claims
- Application Information
AI Technical Summary
Problems solved by technology
Method used
Image
Examples
Embodiment approach
[0109] According to a preferred embodiment of the present invention, the probabilistic structure repair method includes the following steps:
[0110] Step i, obtain the random number of the background of each picture in the pre-training data set in step 1;
[0111] In step ii, set the probability threshold p, and determine whether to introduce additional background by comparing the random number with the probability threshold.
[0112] Among them, in step ii, if the random number of the background of the original picture is greater than the probability threshold p, a new image is sampled from the pre-training data set as the background; if the random number of the background of the original picture is less than or equal to the probability threshold p, the inpainting method is used to repair the background of the original picture .
[0113] Preferably, the probability threshold p ranges from 0 to 1, preferably 0.4.
[0114] In the present invention, the labels of the newly sa...
Embodiment 1
[0145] 1. Dataset
[0146] The method described in the present invention is carried out in COCO and TinyPerson dataset:
[0147] COCO involves 80 classes of objects. The present invention performs network pre-training on a combination of the original training set (including 80k images), the subset (35k images) of the original verification set (Trainval35k) and the 5k subset (Minival) of the verification image.
[0148] TinyPerson is a tiny object detection dataset collected from high-quality videos and web images. In a total of 1,610 images, TinyPerson contains 72,651 low-resolution annotated human objects. 60% and 40% of the images in the dataset are randomly selected as training and testing sets. In TinyPerson, most annotation objects are smaller than 20x20 pixels in size. Subgraphs cut from the origin image are used as input during training and inference prediction. Finally, the results of the same image are combined using the NMS strategy.
[0149] 2. Implementation ...
experiment example 1
[0156] For the scale alignment between the pre-training data set and the target data set, the SM+ method (instance-level scale matching) described in the present invention and the SM method (image-level scale matching) described in the prior art are respectively used for detection, The result is as Figure 4shown.
[0157] Depend on Figure 4 It can be seen that after COCO is adjusted using the SM+ method of the present invention, its aligned distribution is closer to the distribution of TinyPerson. The SM+ method achieves more accurate scale matching, which can achieve higher detection performance.
[0158] Further, the Jensen-Shannon divergence was used to quantitatively measure the similarity between the scale distribution and the target distribution aligned by the RSM+ method, the MSM+ method of the present invention, and the RSM and MAM methods in the prior art.
[0159] Among them, the Jensen-Shannon divergence is a deformation of the Kullback-Leibler divergence, whic...
PUM
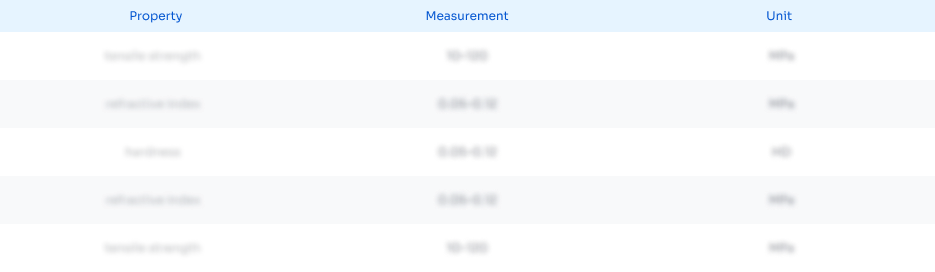
Abstract
Description
Claims
Application Information
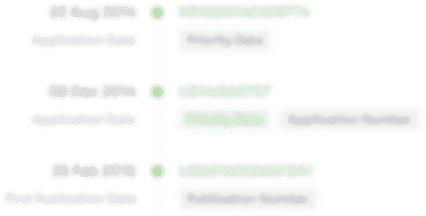
- R&D Engineer
- R&D Manager
- IP Professional
- Industry Leading Data Capabilities
- Powerful AI technology
- Patent DNA Extraction
Browse by: Latest US Patents, China's latest patents, Technical Efficacy Thesaurus, Application Domain, Technology Topic, Popular Technical Reports.
© 2024 PatSnap. All rights reserved.Legal|Privacy policy|Modern Slavery Act Transparency Statement|Sitemap|About US| Contact US: help@patsnap.com