Diabetic retinopathy classification system based on uncertainty
A technology of diabetic retina and classification system, applied in the field of diabetic retinopathy classification system, can solve the problem of inability to display the credibility of model classification results, and achieve the effect of safe and reliable clinical use and guaranteed safety performance.
- Summary
- Abstract
- Description
- Claims
- Application Information
AI Technical Summary
Problems solved by technology
Method used
Image
Examples
Embodiment 1
[0064] Such as figure 1 As shown, the present invention provides a diabetic retinopathy classification system based on uncertainty, the system includes: a storage module for storing images, a preprocessing module, an exudation segmentation module, a microvascular tumor segmentation module, and a classification module;
[0065] The preprocessing module is used for preprocessing the fundus image; it is also used for normalizing the fused multi-channel image of the exudation segmented image, the microvascular tumor segmented image and the corresponding preprocessed fundus image;
[0066] The exudation segmentation module is used to segment the fundus image into exudation segmentation images through the trained exudation segmentation network model;
[0067] The microvascular tumor segmentation module is used to segment the fundus image into microvascular tumor segmentation images through the trained microvascular tumor segmentation network model;
[0068] The classification modul...
PUM
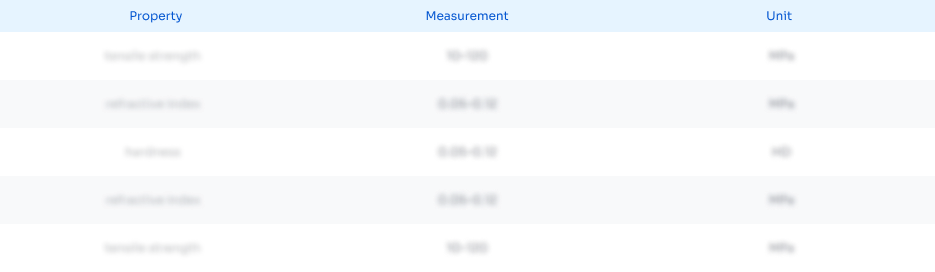
Abstract
Description
Claims
Application Information
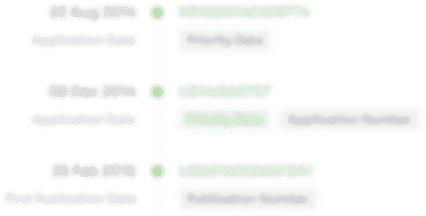
- R&D Engineer
- R&D Manager
- IP Professional
- Industry Leading Data Capabilities
- Powerful AI technology
- Patent DNA Extraction
Browse by: Latest US Patents, China's latest patents, Technical Efficacy Thesaurus, Application Domain, Technology Topic, Popular Technical Reports.
© 2024 PatSnap. All rights reserved.Legal|Privacy policy|Modern Slavery Act Transparency Statement|Sitemap|About US| Contact US: help@patsnap.com