Flexible separable convolution framework, feature extraction method and application thereof in VGG and ResNet
A convolution and frame technology, applied in the field of image processing, can solve problems such as difficulties in actual deployment, difficulty in meeting low-latency practical application requirements, long model reasoning time, etc., to reduce filter depth, reduce information loss, and reduce calculation costs Effect
- Summary
- Abstract
- Description
- Claims
- Application Information
AI Technical Summary
Problems solved by technology
Method used
Image
Examples
Embodiment 1
[0037] This embodiment provides a flexible and separable deep learning convolution framework. On the premise of ensuring accurate operation, this module improves network performance, reduces calculation load, and reduces network parameters.
[0038] Such as figure 1 As shown, a flexible and separable deep learning convolution framework provided in this embodiment includes a feature map clustering and division module, a first convolution operation module, a second convolution operation module, a feature map fusion module, and an attention mechanism SE module , M input channels and N output channels.
[0039] The feature map clustering and dividing module is to divide the M input feature maps into feature maps representing main information and feature maps representing supplementary information according to the proportion of hyperparameter supplementary feature information α, and the proportion of supplementary feature information α is a defined super Parameter, α∈(0,1). In thi...
Embodiment 2
[0050] Such as figure 2 As shown, this embodiment provides a VGG convolutional neural network, including a convolutional layer, a pooling layer, and a fully connected layer. This embodiment only changes the structure of the convolutional layer, and changes the convolutional layer to Embodiment 1. The provided convolutional framework, the VGG convolutional neural network in this embodiment is called FSConv_VGG.
Embodiment 3
[0052] Such as image 3 As shown, this embodiment provides a ResNet-20 network, including a residual block, and the residual block includes a first convolutional layer and a second convolutional layer connected in sequence, and the first convolutional layer and the second convolutional layer The convolution layer adopts the convolution framework provided by Embodiment 1 with the same structure. This embodiment introduces the hyperparameter channel scaling factor β in the output channel of the first convolution layer and the input channel of the second convolution layer, and the value of the channel scaling factor β is Depends on device memory and computing resources; this ResNet-20 network is called FSBneck_ResNet-20.
[0053] Replace the original convolutional layer of VGG-16 and the original residual module of ResNet-20 with Example 2 and Example 3, and verify the results provided by Example 1 on different public datasets (CIFAR-10 and CIFAR-100). Effectiveness of convoluti...
PUM
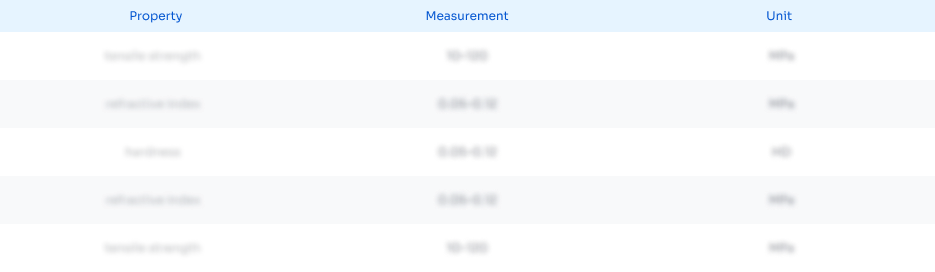
Abstract
Description
Claims
Application Information
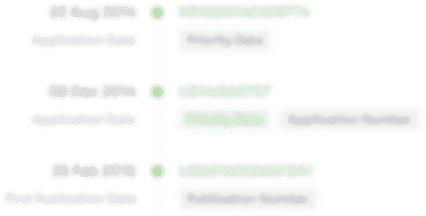
- R&D Engineer
- R&D Manager
- IP Professional
- Industry Leading Data Capabilities
- Powerful AI technology
- Patent DNA Extraction
Browse by: Latest US Patents, China's latest patents, Technical Efficacy Thesaurus, Application Domain, Technology Topic, Popular Technical Reports.
© 2024 PatSnap. All rights reserved.Legal|Privacy policy|Modern Slavery Act Transparency Statement|Sitemap|About US| Contact US: help@patsnap.com