College poor student accurate subsidy model based on LSTM neural network
A neural network and technology for impoverished students, which is applied in the field of precision funding models for impoverished students in colleges and universities, can solve the problems of rare research reports, the precise identification of proposed funding objects needs further research, and the slow convergence speed of BP network, etc., so as to improve fairness and efficiency Effect
- Summary
- Abstract
- Description
- Claims
- Application Information
AI Technical Summary
Problems solved by technology
Method used
Image
Examples
Embodiment
[0101] 1. Data collection:
[0102]The reason why the artificial neural network can simulate the human brain for accurate classification and recognition is that it requires a large amount of data to train and test the neural network, so that the neural network model can classify unknown data patterns. As for the funding work of poor students in colleges and universities, it is necessary to analyze and predict the poverty level of poor students to determine the level of funding they should receive. The consumption data in the all-in-one card can directly reflect the economic status of the students, while the national bursary has the widest range of funding objects, and the formed data is relatively rich, which is suitable for the training and testing of the ANN model. In the present invention, the consumption records of the student campus card of Chongqing Business Vocational College in 2019 are collected, and the attached image 3 Shown are some student consumption records, a...
PUM
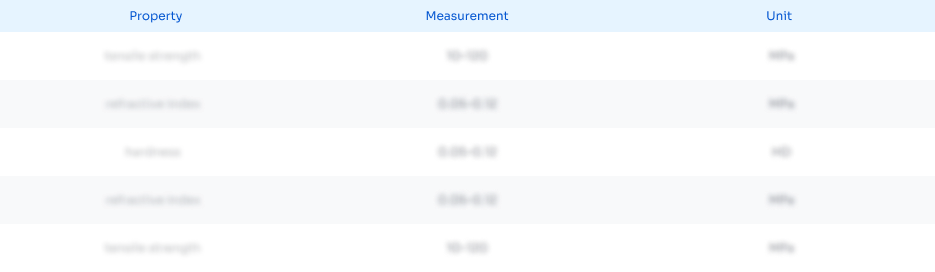
Abstract
Description
Claims
Application Information
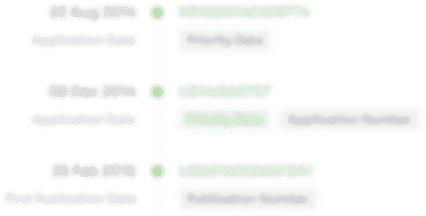
- R&D Engineer
- R&D Manager
- IP Professional
- Industry Leading Data Capabilities
- Powerful AI technology
- Patent DNA Extraction
Browse by: Latest US Patents, China's latest patents, Technical Efficacy Thesaurus, Application Domain, Technology Topic.
© 2024 PatSnap. All rights reserved.Legal|Privacy policy|Modern Slavery Act Transparency Statement|Sitemap