Remote sensing image scene accurate classification method based on Dual-ResNet small sample learning
A technology of remote sensing image and classification method, applied in the field of image processing, can solve problems such as increased computing time, uneven distribution, disappearance of gradient, etc., to reduce computing requirements and resource consumption, avoid the impact of data constraints, and shorten training time. Effect
- Summary
- Abstract
- Description
- Claims
- Application Information
AI Technical Summary
Problems solved by technology
Method used
Image
Examples
Embodiment Construction
[0050] The technical solutions of the present invention will be further described below in conjunction with the accompanying drawings and embodiments.
[0051] like figure 1 As shown, the remote sensing image scene accurate classification method based on Dual-ResNet small sample learning of the present invention specifically includes the following steps:
[0052] (1) Construct a remote sensing scene image sample dataset, and make a corresponding sample category label set, and divide the remote sensing scene image sample dataset into a training set Train and a test set Test. In this embodiment, a public data set of remote sensing scene images UCMerced_LandUse is selected for experimentation, and the data set includes 21 types of remote sensing scene images.
[0053] The method of constructing sample collection and collection classification is as follows:
[0054] (1.1) Construct remote sensing scene image sample dataset Image=[Image 1 ,...,Image i ,...,Image N ], and make ...
PUM
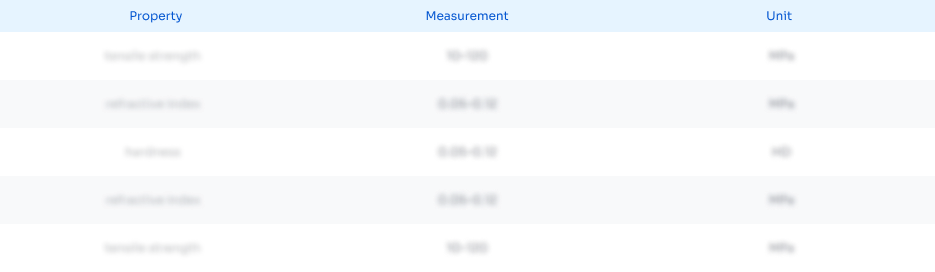
Abstract
Description
Claims
Application Information
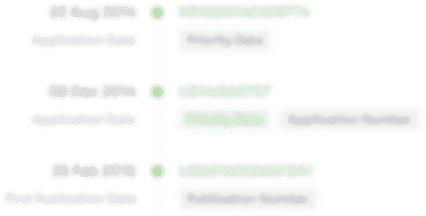
- R&D
- Intellectual Property
- Life Sciences
- Materials
- Tech Scout
- Unparalleled Data Quality
- Higher Quality Content
- 60% Fewer Hallucinations
Browse by: Latest US Patents, China's latest patents, Technical Efficacy Thesaurus, Application Domain, Technology Topic, Popular Technical Reports.
© 2025 PatSnap. All rights reserved.Legal|Privacy policy|Modern Slavery Act Transparency Statement|Sitemap|About US| Contact US: help@patsnap.com