Engine surge fault prediction system and method based on fusion neural network model
A neural network model and fault prediction technology, applied in biological neural network models, neural learning methods, neural architectures, etc., can solve the problems of limited fault modes, time-consuming and labor-intensive prediction accuracy, and low prediction accuracy, so as to achieve rapid prediction and improve accuracy , Improving the effect of precision and recall
- Summary
- Abstract
- Description
- Claims
- Application Information
AI Technical Summary
Problems solved by technology
Method used
Image
Examples
Embodiment 1
[0053] Such as figure 1 As shown, in Embodiment 1, the engine surge fault prediction system based on the fusion neural network model, the system based on the fusion neural network (PCFNN) of the present invention specifically includes a sequentially connected prediction module, feature extraction module and classification module. Specifically, the prediction module is used to generate a forecast time series of a specified length from the three-dimensional structure time series data of the engine; the feature extraction module is used to extract the local features of the forecast time series, the semantic relationship between data, and the overall sequence trend feature; the classification module, It is used to judge whether it is a surge fault based on the local characteristics of the predicted time series, the semantic relationship between data, and the overall sequence trend characteristics. The system prediction module of the present invention generates the three-dimensiona...
Embodiment 2
[0069] This embodiment has the same inventive concept as Embodiment 1. On the basis of the embodiment, a method for predicting engine surge faults based on a fusion neural network model is provided. The method includes the following steps:
[0070] S1: Generating the three-dimensional structural time series data of the engine into a predicted time series of a specified length;
[0071] S2: Extract the local features of the predicted time series, the semantic relationship between the data, and the overall sequence trend features;
[0072] S3: Determine whether it is a surge fault based on the local characteristics of the predicted time series, the semantic relationship between the data, and the overall sequence trend characteristics.
[0073]Further, in step S1, generating a forecast time series of a specified length from the three-dimensional structural time series data of the engine specifically includes:
[0074] S11: Encode the three-dimensional structural time series data...
Embodiment 3
[0095] This embodiment provides a storage medium, which has the same inventive concept as that of Embodiment 2, on which computer instructions are stored, and when the computer instructions are run, the engine surge fault prediction method based on the fusion neural network model in Embodiment 2 is executed. step.
[0096] Based on this understanding, the technical solution of this embodiment is essentially or the part that contributes to the prior art or the part of the technical solution can be embodied in the form of a software product, and the computer software product is stored in a storage medium. Several instructions are included to make a computer device (which may be a personal computer, a server, or a network device, etc.) execute all or part of the steps of the methods in various embodiments of the present invention. The aforementioned storage medium includes: U disk, mobile hard disk, read-only memory (Read-Only Memory, ROM), random access memory (Random Access Mem...
PUM
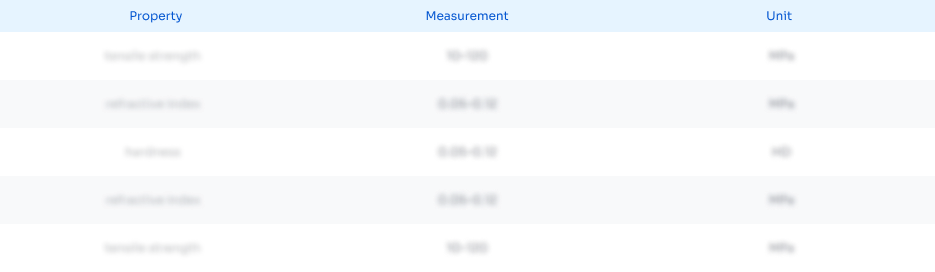
Abstract
Description
Claims
Application Information
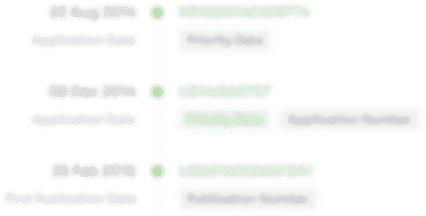
- R&D
- Intellectual Property
- Life Sciences
- Materials
- Tech Scout
- Unparalleled Data Quality
- Higher Quality Content
- 60% Fewer Hallucinations
Browse by: Latest US Patents, China's latest patents, Technical Efficacy Thesaurus, Application Domain, Technology Topic, Popular Technical Reports.
© 2025 PatSnap. All rights reserved.Legal|Privacy policy|Modern Slavery Act Transparency Statement|Sitemap|About US| Contact US: help@patsnap.com