Multi-task learning model construction and optimization method based on deformable convolution
A technology of multi-task learning and construction method, applied in the field of multi-task learning model construction and optimization based on deformable convolution, can solve problems such as limited spatial modeling ability and inability to extract distinguishing specific task features, and achieve enhanced Spatial modeling capabilities, promoting the optimization of difficult tasks, and expanding the effect of the receptive field
- Summary
- Abstract
- Description
- Claims
- Application Information
AI Technical Summary
Problems solved by technology
Method used
Image
Examples
Embodiment 1
[0040] This embodiment is designed from the aspect of the network structure design of the multi-task learning model, which significantly enhances the spatial modeling transformation capability of the multi-task learning model, and extracts and mines more distinguishing specific task features.
[0041] In the present invention, the multi-task learning model construction method based on deformable convolution introduces deformable convolution into multi-task learning when designing the network structure of the multi-task learning model. Such as figure 1 As shown, firstly, the feature sharing network is used to extract feature sharing blocks at different levels; the deformable convolutional layer and the feature alignment layer are sequentially connected to build a task-specific deformable module; finally, the task-specific deformable module is shared with different levels of feature blocks Directly corresponding to the connection, in this process, the self-adaptive adjustment is...
Embodiment 2
[0064] The construction of the multi-task network model is completed through the steps described in Embodiment 1, and then the multi-task optimization design is carried out in this embodiment.
[0065] This embodiment provides a multi-task learning model optimization method based on deformable convolution, using the weight reset method for multi-task optimization: in the training process, the difficulty of the sub-tasks is ranked in real time according to the relative loss reduction rate, and the The weight of the loss function of the easiest subtask is reset to zero, and the remaining subtasks continue to train; after two rounds of iterations, restore the weight parameters that were set to zero; repeat the above steps to achieve a dynamic balance between subtasks during the training process, so that all subtasks tasks are of equal importance.
[0066] Specifically include the following steps:
[0067] Step 5: Subtask weight initialization: According to the complexity of the ...
PUM
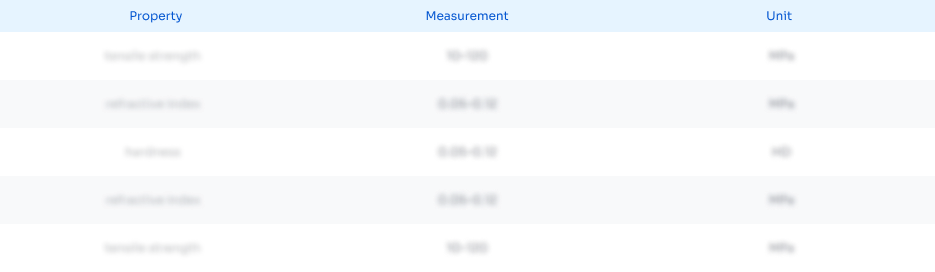
Abstract
Description
Claims
Application Information
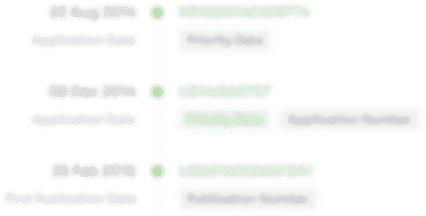
- R&D Engineer
- R&D Manager
- IP Professional
- Industry Leading Data Capabilities
- Powerful AI technology
- Patent DNA Extraction
Browse by: Latest US Patents, China's latest patents, Technical Efficacy Thesaurus, Application Domain, Technology Topic, Popular Technical Reports.
© 2024 PatSnap. All rights reserved.Legal|Privacy policy|Modern Slavery Act Transparency Statement|Sitemap|About US| Contact US: help@patsnap.com