Pedestrian re-recognition method based on multi-task learning
A pedestrian re-identification and multi-task learning technology, applied in the field of pedestrian re-identification and pedestrian re-identification based on multi-task learning, can solve the problems of reducing robustness, learning and matching, and a large amount of labeled data, so as to make up for defects and improve Performance, the effect of simplifying training
- Summary
- Abstract
- Description
- Claims
- Application Information
AI Technical Summary
Problems solved by technology
Method used
Image
Examples
Embodiment Construction
[0045] The technical solution of the present invention will be further described in detail below in conjunction with the accompanying drawings.
[0046] Such as figure 1 A pedestrian re-identification method based on multi-task learning is shown, including the following steps.
[0047]Step 1) Obtain cross-camera pedestrian images, construct a pedestrian re-identification training data set, the data set contains a preset number of pedestrian images;
[0048] In this embodiment, the Gaussian mixture model is used to detect the foreground of the pedestrian image; according to the detection result of the Gaussian mixture model, if there is a video frame of the moving foreground, a pre-trained pedestrian detector is used to detect the pedestrian, accurately locate the pedestrian position, and from The image of the corresponding area is intercepted in the video frame as the pedestrian image; if the Gaussian mixture model does not detect the moving foreground, the pedestrian detecto...
PUM
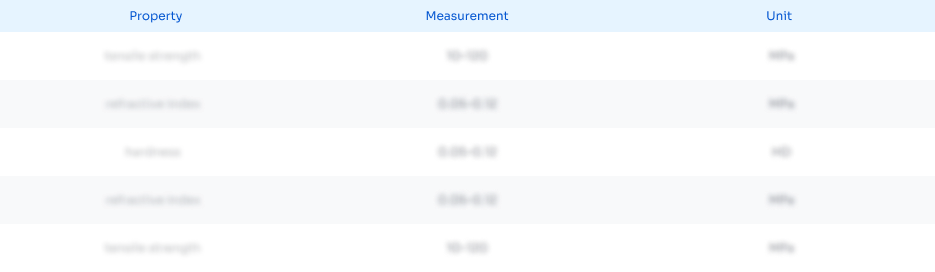
Abstract
Description
Claims
Application Information
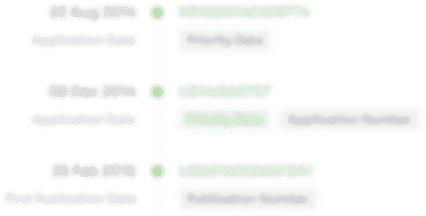
- R&D Engineer
- R&D Manager
- IP Professional
- Industry Leading Data Capabilities
- Powerful AI technology
- Patent DNA Extraction
Browse by: Latest US Patents, China's latest patents, Technical Efficacy Thesaurus, Application Domain, Technology Topic, Popular Technical Reports.
© 2024 PatSnap. All rights reserved.Legal|Privacy policy|Modern Slavery Act Transparency Statement|Sitemap|About US| Contact US: help@patsnap.com