Hyperspectral image super-resolution optimization method based on deep closed-loop neural network
A hyperspectral image and neural network technology, applied in neural learning methods, biological neural network models, neural architectures, etc., can solve problems such as difficult learning of mapping relationships, limited data, and inability to include all ground objects, etc., to achieve reduced mapping space, good reconstruction results, and reduced adverse effects
- Summary
- Abstract
- Description
- Claims
- Application Information
AI Technical Summary
Problems solved by technology
Method used
Image
Examples
Embodiment Construction
[0014] Such as figure 1 Shown, a kind of satellite hyperspectral image super-resolution method based on deep closed-loop neural network of the present invention comprises the following steps:
[0015] (1) Establish a deep closed-loop neural network model based on hyperspectral data:
[0016] Step 1. Obtain hyperspectral data from satellites Y gt , simulated with bicubic interpolation to generate Y gt Corresponding low-resolution hyperspectral image ;
[0017] Step 2. Establish the structure of the deep closed-loop neural network, including the super-resolution neural network model and the inverse super-resolution neural network model, set the parameters, and input Y gt and X lr image pairs to train the model.
[0018] (2) Reconstruction of high-resolution hyperspectral images by variable separation fine optimization method:
[0019] Step 3. Construct an objective function including data items and prior items according to the trained deep closed-loop neural network,...
PUM
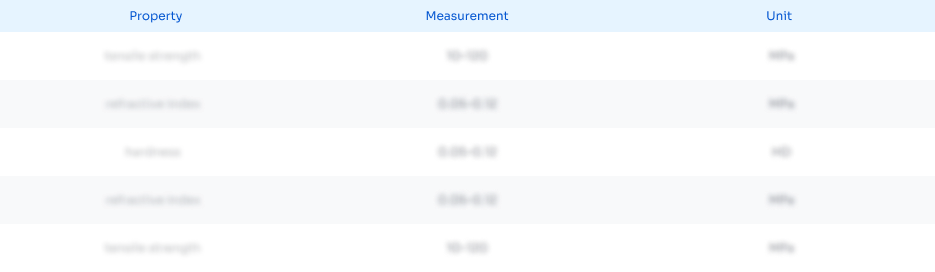
Abstract
Description
Claims
Application Information
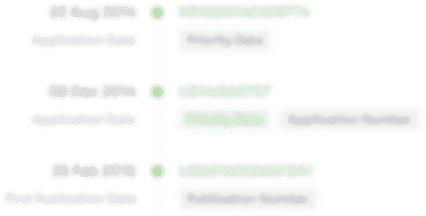
- R&D Engineer
- R&D Manager
- IP Professional
- Industry Leading Data Capabilities
- Powerful AI technology
- Patent DNA Extraction
Browse by: Latest US Patents, China's latest patents, Technical Efficacy Thesaurus, Application Domain, Technology Topic, Popular Technical Reports.
© 2024 PatSnap. All rights reserved.Legal|Privacy policy|Modern Slavery Act Transparency Statement|Sitemap|About US| Contact US: help@patsnap.com